Pair People and AI for Better Product Demand Forecasting
A new framework helps leaders orchestrate human and AI agents to accurately forecast product demand.
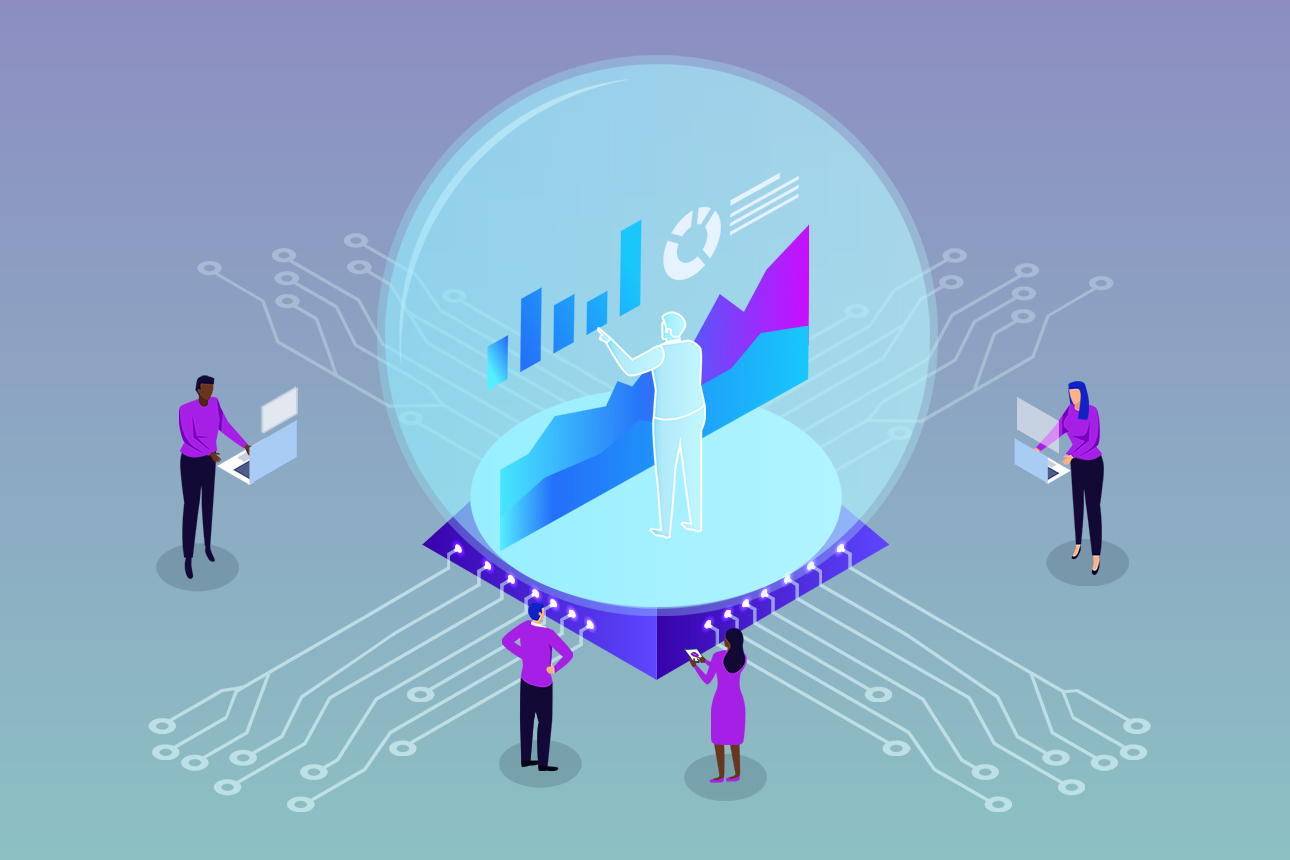
Carolyn Geason-Beissel/MIT SMR | Getty Images
Hot pink made a comeback this past summer. But as numerous fashion brands eagerly jumped on the Barbie-driven trend, many struggled to get their numbers right. Aldo, an official brand collaborator, saw its Barbie-based platform shoes fly off the shelves within 24 hours, thanks to the viral hashtag #TikTokMadeMeBuyIt.
The Barbie phenomenon highlights a modern dilemma: how to accurately forecast product demand when the commercial landscape is constantly changing. This has always been a challenge, but the retail environment is now more volatile and fad-driven than it was in the past, requiring companies to take demand forecasting to a higher level.
Many companies have implemented next-generation tools like artificial intelligence to meet the challenge. While algorithms are improving forecasting performance, human interventions are still needed to contextualize market changes and bring other attributes, like responsiveness, to the table.1 Because there’s no standard template yet for such relationships, we have developed a framework to help companies marry human expertise with AI-driven forecasting solutions, based on a product’s characteristics.
Product Complexities
One hurdle to developing demand forecasting models today is the widely varying characteristics of different product categories. Short life cycle products, such as those in the fashion, beauty, and high-tech industries, have little to no historical data, making forecasting demand a challenging task. These products also pose a higher risk to companies because they are susceptible to higher stockouts or excess stock levels, as seen in the case of the Barbie merchandise.
References
1. See E. Revilla, M.J. Saenz, M. Seifert, et al., “Human-Artificial Intelligence Collaboration in Prediction: A Field Experiment in the Retail Industry,” Journal of Management Information Systems 40, no. 4 (December 2023): 1071-1098.
2. K. Hu, J. Acimovic, F. Erize, et al., “Forecasting New Product Life Cycle Curves: Practical Approach and Empirical Analysis,” Manufacturing & Service Operations Management 21, no. 1 (winter 2019): 66-85.
3. X. Li, Y. Yin, D.V. Manrique, et al., “Lifecycle Forecast for Consumer Technology Products With Limited Sales Data,” International Journal of Production Economics 239 (September 2021): 1-10.