Why So Many Data Science Projects Fail to Deliver
Organizations can gain more business value from advanced analytics by recognizing and overcoming five common obstacles.
Topics
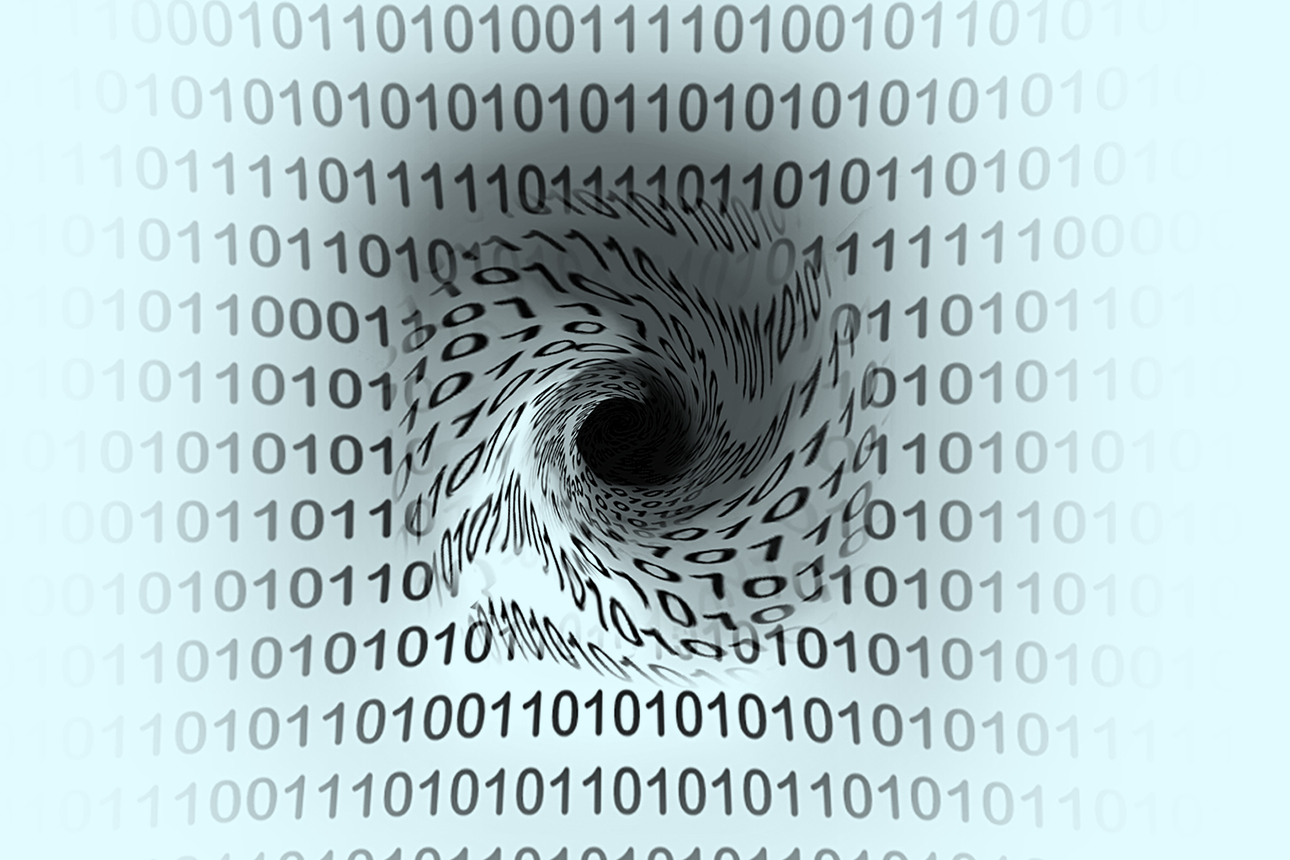
Image courtesy of Jean Francois Podevin/theispot.com
More and more companies are embracing data science as a function and a capability. But many of them have not been able to consistently derive business value from their investments in big data, artificial intelligence, and machine learning.1 Moreover, evidence suggests that the gap is widening between organizations successfully gaining value from data science and those struggling to do so.2
To better understand the mistakes that companies make when implementing profitable data science projects, and discover how to avoid them, we conducted in-depth studies of the data science activities in three of India’s top 10 private-sector banks with well-established analytics departments. We identified five common mistakes, as exemplified by the following cases we encountered, and below we suggest corresponding solutions to address them.
Get Updates on Leading With AI and Data
Get monthly insights on how artificial intelligence impacts your organization and what it means for your company and customers.
Please enter a valid email address
Thank you for signing up
Mistake 1: The Hammer in Search of a Nail
Hiren, a recently hired data scientist in one of the banks we studied, is the kind of analytics wizard that organizations covet.3 He is especially taken with the k-nearest neighbors algorithm, which is useful for identifying and classifying clusters of data. “I have applied k-nearest neighbors to several simulated data sets during my studies,” he told us, “and I can’t wait to apply it to the real data soon.”
Hiren did exactly that a few months later, when he used the k-nearest neighbors algorithm to identify especially profitable industry segments within the bank’s portfolio of business checking accounts. His recommendation to the business checking accounts team: Target two of the portfolio’s 33 industry segments.
This conclusion underwhelmed the business team members. They already knew about these segments and were able to ascertain segment profitability with simple back-of-the-envelope calculations. Using the k-nearest neighbors algorithm for this task was like using a guided missile when a pellet gun would have sufficed.
References
1. R. Bean and T.H. Davenport, “Companies Are Failing in Their Efforts to Become Data-Driven,” Harvard Business Review, Feb. 5, 2019, https://hbr.org.
2. T.H. Davenport, N. Mittal, and I. Saif, “What Separates Analytical Leaders From Laggards?” MIT Sloan Management Review, Feb. 3, 2020, https://dev03.mitsmr.io.
3. The names of people and organizations are pseudonyms, in keeping with our agreements with the companies.
4. S. Ransbotham, “Deodorizing Your Data,” MIT Sloan Management Review, Aug. 24, 2015, https://dev03.mitsmr.io.
5. T. O’Toole, “What’s the Best Approach to Data Analytics?” Harvard Business Review, March 2, 2020, https://hbr.org.
6. S. Ransbotham, “Avoiding Analytical Myopia,” MIT Sloan Management Review, Jan. 25, 2016, https://dev03.mitsmr.io.
7. P. Puranam, M. Raveendran, and T. Knudsen, “Organization Design: The Epistemic Interdependence Perspective,” Academy of Management Review 37, no. 3 (July 2012): 419-440.
Comments (2)
S Obi
Shankar Hn