Why ‘Explicit Uncertainty’ Matters for the Future of Ethical Technology
What if algorithms were built around users’ objectives rather than the company’s end goals?
Topics
Developing an Ethical Technology Mindset
Brought to you by
Deloitte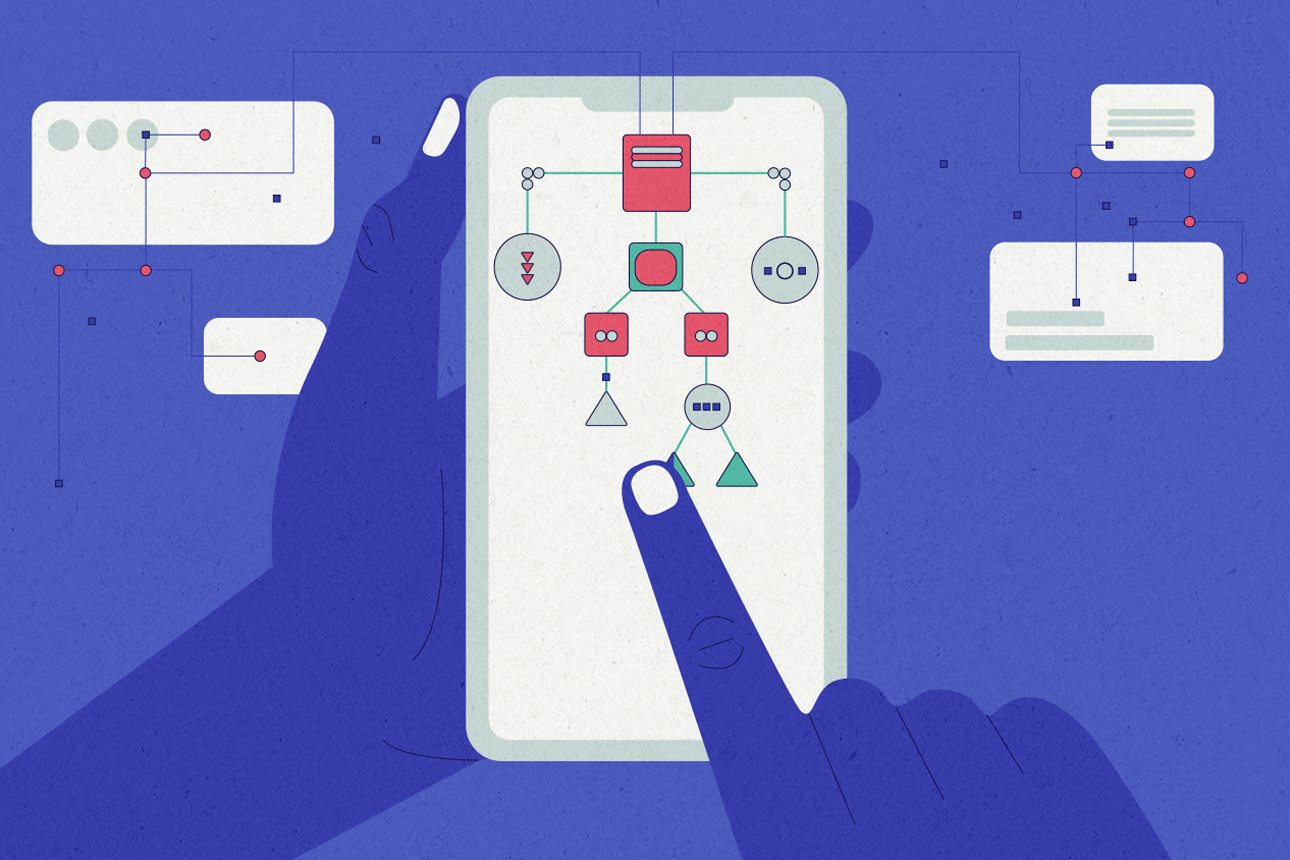
Image courtesy of Laura Wentzel
The biggest concerns over AI today are not about dystopian visions of robot overlords controlling humanity. Instead, they’re about machines turbocharging bad human behavior. Social media algorithms are one of the most prominent examples.
Take YouTube, which over the years has implemented features and recommendation engines geared toward keeping people glued to their screens. As The New York Times reported in 2019, many content creators on the far right learned that they could tweak their content offerings to make them more appealing to the algorithm and drive many users to watch progressively more extreme content. YouTube has taken action in response, including efforts to remove hate speech. An independently published study in 2019 claimed that YouTube’s algorithm was doing a good job of discouraging viewers from watching “radicalizing or extremist content.” Still, as recently as July 2021, new research found that YouTube was still sowing division and helping to spread harmful disinformation.
Twitter and Facebook have faced similar controversies. They’ve also taken similar steps to address misinformation and hateful content. But the initial issue remains: The business objective is to keep users on the platform. Some users and content creators will take advantage of these business models to push problematic content.
Algorithms like YouTube’s recommendation engine are programmed with an end goal: engagement. Here, machine learning adapts and optimizes based on user behavior to accomplish that goal. If certain content spurs higher engagement, the algorithm may naturally recommend that same content to other people, all in service of that goal.
This can have far-ranging effects for society. As Sen. Chris Coons of Delaware put it in April 2021 when executives from YouTube, Facebook, and Twitter were testifying before Congress, “These algorithms are amplifying misinformation, feeding political polarization, and making us more distracted and isolated.”
To address this issue, companies and leaders must consider the ethical implications of technology-driven business models. In the example of social media, how differently might an algorithm work if it instead had no end goal?
Avoiding Fixed Objectives
In a report for the Center for Human-Compatible AI, we call for a new model for AI. It’s built around what may seem like a radical idea: explicit uncertainty. Using this model, the algorithm has no intrinsic objective.