When You Reject People, Tell Them Why
Explainable AI and ethical human judgment both play important roles in fair, accurate talent assessment.
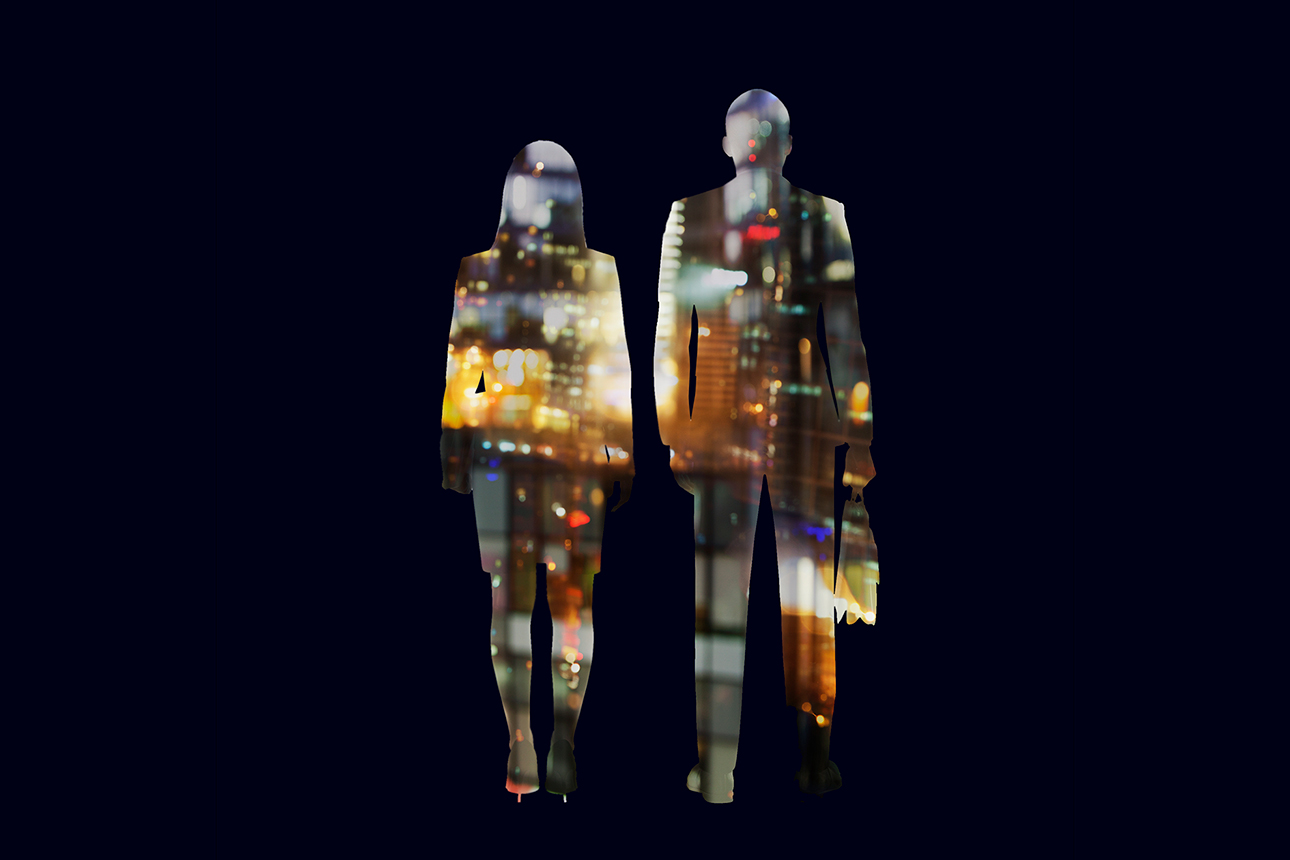
Humans are meaning-making machines, continually searching for patterns and creating stories to make sense of them. This intense desire to understand the world goes hand in hand with the rise of artificial intelligence in organizations. We expect AI to advance our quest for meaning — not just to predict that X leads to Y in the workplace but to shed light on the reason. In short, we expect it to be explainable.
Definitions vary, but in a recent academic paper, my colleagues and I described explainable AI as “the quality of a system to provide decisions or suggestions that can be understood by their users and developers.” That’s important for applications designed to evaluate people.
For example, most hiring managers are not content knowing that an algorithm selected a certain person for a job or that someone “did well” on a video interview where AI was used as the scoring engine. They also want to know in what ways people performed well: Did they make more eye contact than others? Were they less sweaty and fidgety? Did they use more words with emotional impact? Of course, the candidates want to know those things too. Otherwise, the results feel arbitrary and nothing can be learned and applied to the next job application or interview.
In the early days of the AI revolution, companies were excited about their new window into employee behavior: If someone, say, went to the bathroom more than three times a day (at work, that is — back when more of us worked in an office), they were deemed X% more likely to leave their job. But such insights about people can only be described as pointless — unless we can qualify them by saying that those who left were (a) stressed, (b) bored, or (c) fired for doing drugs in the bathroom. That’s a hypothetical range of options, but the point is that any explanation is better than no explanation. This is something scientists have known for ages: To go from data to insights, you need context or, even better, a model. Science is data plus theory, and that’s just as true when we’re assessing people as when we’re assessing ideas. Why matters.
Transparent Tools
Explainable AI sounds like a new concept, but in essence it has been around for decades. For example, in the U.S.,
Comment (1)
Jeff Schwartz