Want to Make Better Decisions? Start Experimenting
Four lessons for using randomized controlled experiments to create value for your company and customers.
Topics
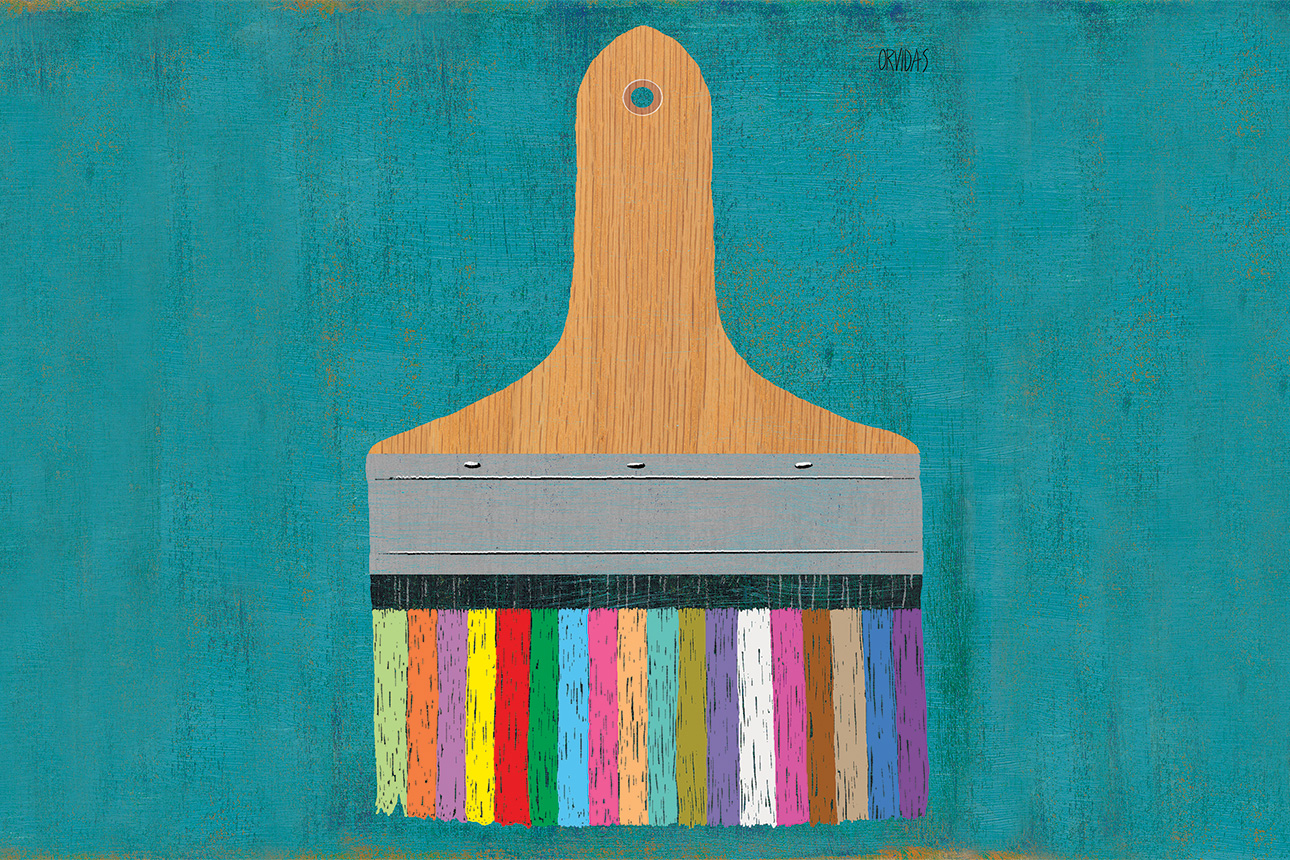
Image courtesy of Ken Orvidas/theispot.com
Suppose you work on Google’s advertising team and need to decide whether ads should have a blue background or a yellow background. You think that yellow would attract the most clicks; your colleague thinks that blue is better. How do you make the decision?
In Google’s early days, the two of you might have debated the issue until someone caved or you both agreed to kick the decision up to the boss. But ultimately, it dawned on leaders throughout Google that many of these debates and decisions were unnecessary.
“We don’t want high-level executives discussing whether a blue background or a yellow background will lead to more ad clicks,” Hal Varian, Google’s chief economist, told us. “Why debate this point, since we can simply run an experiment to find out?”
Varian worked with the team that developed Google’s systematic approach to experimentation. The company now runs experiments at an extraordinary scale — more than 10,000 per year. The results of these experiments inform managerial decisions in a variety of contexts, ranging from advertising sales to search engine parameters.
More broadly, an experimental mindset has permeated much of the tech sector and is spreading beyond that. These days, most major tech companies, such as Amazon, Facebook, Uber, and Yelp, wouldn’t make an important change to its platforms without running experiments to understand how it might influence user behavior. Some traditional businesses, such as Campbell Soup Co., have been dipping their toes into experiments for decades.1 And many more are ramping up their efforts in experimentation as they undergo digital transformations. In a dramatic departure from its historic role as an esoteric tool for academic research, the randomized controlled experiment has gone mainstream. Startups, international conglomerates, and government agencies alike have a new tool to test ideas and understand the impact of the products and services they are providing.
Experiments complement intuition and guesswork with evidence-based insight. We’ve seen them lead to large returns for organizations — such as when an experiment alerted eBay that it was wasting millions of advertising dollars per year. (See “The Returns on Experimentation.
References
1. J.O. Eastlack, Jr. and A.G. Rao, “Advertising Experiments at the Campbell Soup Company,” Marketing Science 8, no. 1 (February 1989): 57-71.
2. R. Meyer, “Everything We Know About Facebook’s Secret Mood Manipulation Experiment,” The Atlantic, June 28, 2014, www.theatlantic.com.
3. T. Blake, S. Moshary, K. Sweeney, et al., “Price Salience and Product Choice,” working paper 25186, National Bureau of Economic Research, Cambridge, Massachusetts, October 2018.
4. R. Kaufman, J. Pitchforth, and L. Vermeer, “Democratizing Online Controlled Experiments at Booking.com” (presentation at the MIT Conference on Digital Experimentation, Cambridge, Massachusetts, Oct. 27-28, 2017).
5. D.J. Zhang, H. Dai, L. Dong, et al., “How Do Price Promotions Affect Customer Behavior on Retailing Platforms? Evidence From a Large Randomized Experiment on Alibaba,” Management Science 27, no. 12 (December 2018): 2343-2345.
6. “The Handbook of Experimental Economics,” eds. J.H. Kagel and A.E. Roth (Princeton, New Jersey: Princeton University Press, 1995).
7. More information on Behavior Change for Good’s research and experiments is available at https://bcfg.wharton.upenn.edu.
8. A.D.I. Kramer, J.E. Guillory, and J.T. Hancock, “Experimental Evidence of Massive-Scale Emotional Contagion Through Social Networks,” Proceedings of the National Academy of Sciences 111, no. 24 (June 17, 2014): 8788-8790.
9. Z. Boka, “Facebook’s Research Ethics Board Needs to Stay Far Away From Facebook,” Wired, June 23, 2016, www.wired.com.
i. M. Ostrovsky and M. Schwarz, “Reserve Prices in Internet Advertising Auctions: A Field Experiment,” working paper 2054, Stanford University Graduate School of Business, Stanford, California, November 2016.
ii. T. Blake, C. Nosko, and S. Tadelis, “Consumer Heterogeneity and Paid Search Effectiveness: A Large-Scale Field Experiment,” Econometrica 83, no. 1 (January 2015): 155-174.
iii. N. Bloom, B. Eifert, A. Mahajan, et al., “Does Management Matter? Evidence From India,” working paper 16658, National Bureau of Economic Research, Cambridge, Massachusetts, January 2011.
iv. E.P. Bettinger, B.T. Long, P. Oreopoulos, et al., “The Role of Application Assistance and Information in College Decisions: Results From the H&R Block Fafsa Experiment,” Quarterly Journal of Economics 127, no. 3 (August 2012): 1205-1242.