Unlocking the Potential of Digital Twins in Supply Chains
As companies optimize a wide range of supply chain functions with digital twins, the technology’s immense potential is gaining further recognition.
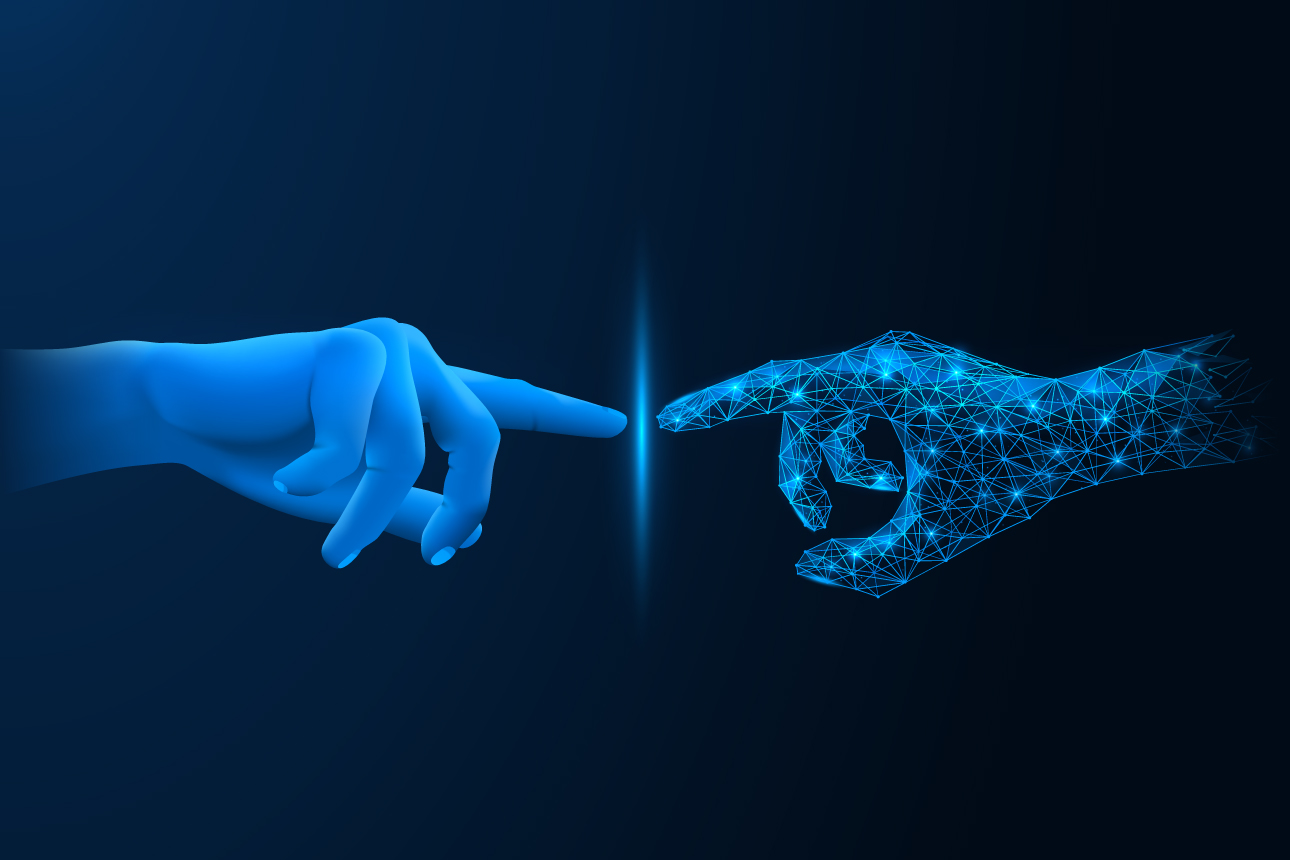
Since the early 2000s, digital twin technology has been adopted across many industries and become more accessible and affordable, yet it remains underutilized in supply chain management. This is partly explained by the complex nature of supply chains themselves and partly due to misunderstandings about the technology’s applications, capabilities, and potential value. However, with a proper adoption and implementation strategy, digital twins can deliver immense benefits across a wide range of supply chains.
Distinguishing Characteristics of Digital Twins
Digital twins — virtual replicas of physical entities and their interactions — consist of a combination of enabling technologies and analytics capabilities. But the technology is often misunderstood; many people incorrectly assume that digital twins are themselves sensors, 3D models, simulators, or applications of AI technology. Others mistakenly consider digital twins to be largely theoretical and not relevant for supply chain management, or assume that a digital twin can be built only after the physical twin has been created — but neither statement is true.
Digital twins are a combination of multiple enabling technologies, such as sensors, cloud computing, AI and advanced analytics, simulation, visualization, and augmented and virtual reality. Companies can use a customized mix of technologies, depending on their needs and expectations. What distinguishes digital twins and makes them so powerful is their ability to emulate human capabilities, support critical decision-making, and even make decisions on behalf of humans.
Digital twins observe their physical environment through a network of sensors that dynamically gather real-time data; they evolve by learning from this information and its contexts and by interacting with humans, devices, and other networked digital twins. Such a capability makes digital twins active and social tools, because they can continuously communicate and collaborate with their associated physical and digital objects and with humans. Digital twins support end-to-end visibility and traceability, enabling supply chain practitioners to spot patterns of highly complex and dynamic behavior.
Digital twins can oversee many internal and external moving parts in end-to-end supply chains and build nonlinear supply chain models. More critically, with their ability to compute thousands of what-if scenarios, the technology learns from these decisions and gains in maturity over time. This helps managers make faster, more accurate, and better-informed decisions with long-term impact at a considerably lower cost.