The Data Science Management Process
Data science initiatives should be integrated with the overall business strategy, and then overseen by an intermediary group that works between the company and its data scientists.
Topics
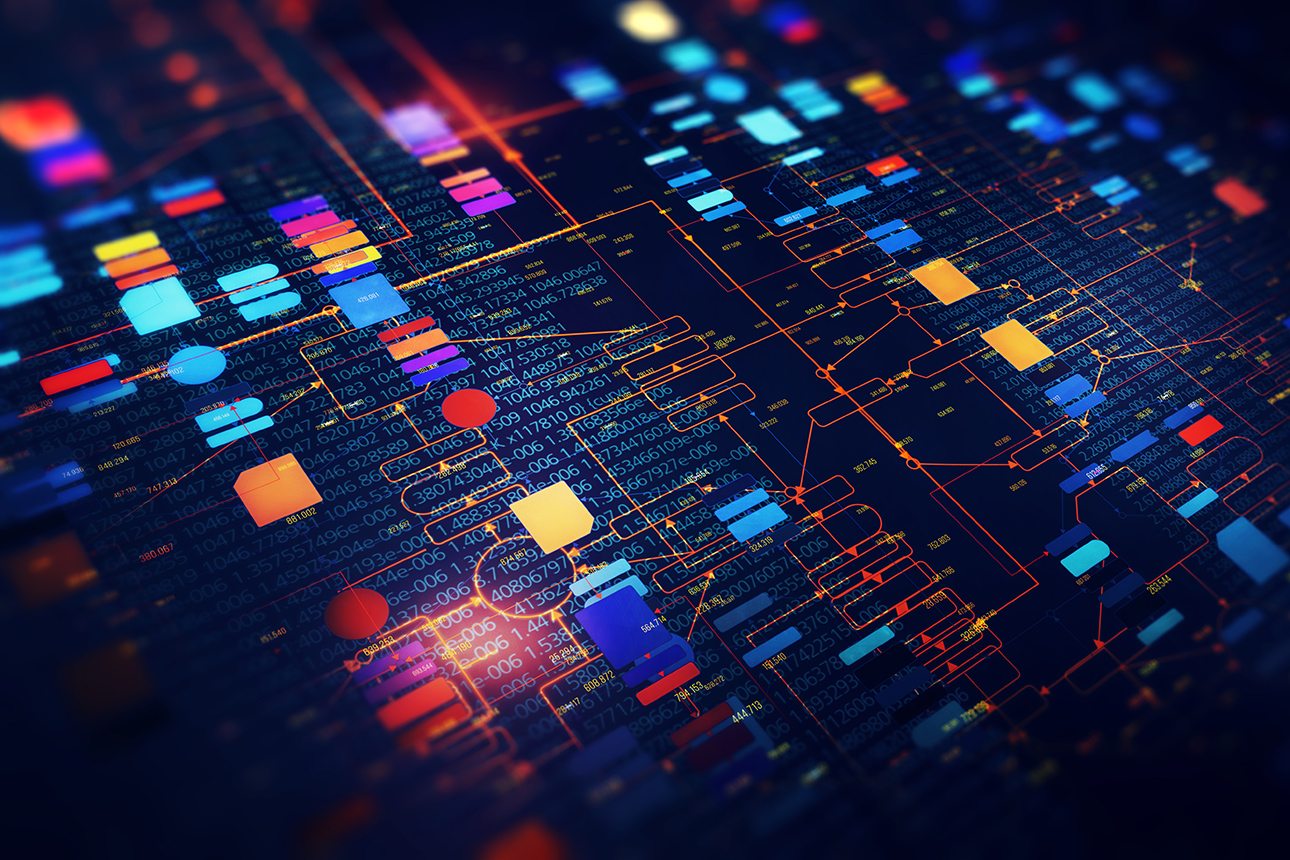
It is increasingly clear that companies and government agencies do not know how to manage data science at the enterprise level. Many are still stuck doing pilots. Some take on projects that are beyond their capabilities. And too often, excellent work dies on the vine during implementation. Companies must take action to address the structural and process issues that hold them back.
In an earlier article, we pointed out the major structural flaw hindering many data science programs — the inherent conflict between data science groups (which we termed the lab) and business operations (termed the factory). To resolve that conflict, we proposed a data science bridge: an intermediary group headed by a person with the title innovation marshal tasked with ensuring better communication and integration between the two groups and surfacing the best ways to make inventions by the lab fit into the needs of the factory.
This article builds on that structural solution by addressing the issues associated with managing the process at an enterprise level. Proper management includes driving collaboration, developing human capital, ensuring data quality, managing the project portfolio, and ensuring the business impact of all data science efforts. We propose that this overall data science management process be owned by the person leading the data science bridge.
Five Core Tasks for Managing Data Science
Managing the process requires, at a minimum, proper organizational structure — that is, the bridge — as well as the right people in place within this structure and the right set of core tasks. (See “Visualizing the Data Science Management Process.”) This model draws on well-established management structures for finance, human resources, manufacturing, and marketing.
Our proposed data science management process is presented as a cycle, or continuous loop. Data science resides within the context of the organization and its overall business strategy. That strategy determines what needs to be accomplished and provides high-level direction to the data science bridge.