Reskilling Talent to Shrink Technology Gaps
Leaders must focus on managing the gaps in AI skills and processes within the organization.
Topics
The AI & Machine Learning Imperative
Brought to you by
AWS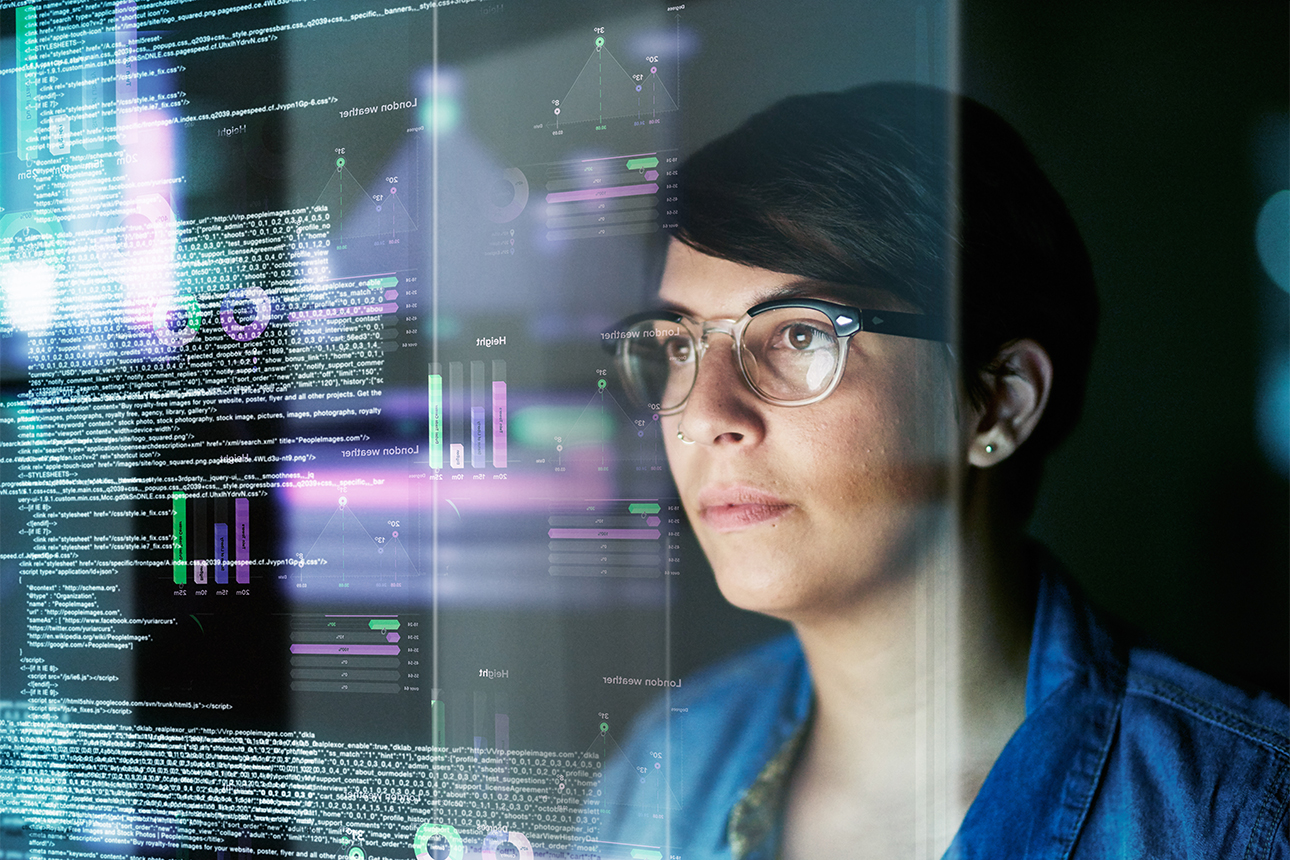
Frustrated with artificial intelligence efforts, the CIO at a large pharma company characterized the products and services from AI vendors as bright but “very young children” requiring tons of effort from internal staff members to reach the maturity to solve practical business problems. The company could buy AI-enabled products and services, but purchasing alone was not enough. Acquiring sophisticated AI technology still left the organization far from achieving strategic goals and increasing business value.
This company’s dilemma isn’t an isolated case. Despite the growing prevalence of AI technology and copious data within companies, getting value from AI isn’t easy. Even with AI technology increasingly easier to acquire, 40% of organizations making significant investments in AI still do not report business gains from AI. As with technology advances in the past, technology alone isn’t the answer to value.
Get Updates on Leading With AI and Data
Get monthly insights on how artificial intelligence impacts your organization and what it means for your company and customers.
Please enter a valid email address
Thank you for signing up
Instead, getting value from AI requires investment beyond technology, notably in data infrastructure and talent. AI talent can be a particularly difficult limitation. Once armed with technology and infrastructure, many organizations find that they don’t have the AI skills they need.
Technology creates an inevitable gap — a gap between the sophisticated solutions an organization produces with a given technology and what portion of that production the organization can use. Spiffy models don’t help if people in the organization don’t know what the results mean or what they should do differently based on the results. The problem for managers, therefore, is less about managing the technology itself and more about managing the skills and processes needed by people and teams.
To illustrate, consider the relationship between the maturity of an organization with a particular technology and the sophistication of its use of that technology. As an organization matures, the technical sophistication likely improves in general. But this technical sophistication isn’t distributed evenly throughout the organization. Some employees have greater technical skills than others do. Some organizational roles (such as AI and IT teams working on the production and development side of the technology) are likely more technically sophisticated than those of employees who consume those results (such as upper management or customer service teams). Compounding the difficulty, as the organization matures, the skill levels among employee groups develop at different rates.
As organizations put more resources into a general-purpose technology such as AI, they can produce more sophisticated results with the technology. (See “Maturation of Technology Sophistication.”) Employees working directly with AI will gain experience. For example, customer churn models can boost prediction through more sophisticated algorithms, fraud detection can better discern legitimate from nefarious transactions, or inventory logistics can continuously refine routing and stock replenishment. All of these applications benefit significantly from recent improvements in AI, increasing the sophistication of AI-based results that the organization has at its disposal.
Fortunately, the rate of increase in production sophistication can improve sharply. Ecosystems scramble to offer products and services to help organizations make the most of technology advances. For example, when an organization doesn’t have the necessary talent to produce results with a technology like AI, it can look outside for help. Unfortunately, outsourcing delivers AI-related value to only 12% of organizations that report using this approach.
This bleak statistic doesn’t necessarily mean that outsourcing doesn’t work. External talent can help organizations improve productivity and results with new technology. Organizations can quickly benefit from the hard-won experience of others without the hardship of winning it themselves. But, as the disappointed pharma executive found, expertise about the specific business context is necessary, particularly as organizations try to apply AI to core, unique processes.
The difficulty is that producing results with AI systems alone isn’t enough. The organization must be able to use these results to further business goals. Salespeople need to understand how to use the customer churn predictions and what they mean to their organization. Customer service agents need to understand why the system flagged a customer’s transaction as fraudulent. Supply chain managers need to know why a system recommends a particular production level or logistics plan. It asks a lot of experienced workers to trust algorithmic results that they don’t yet understand.
Yes, organizations can increase their consumption of new technology results and data. And companies and managers can help employees improve their ability to work alongside machines. Consumption improvements are necessary for companies to see the benefits of new, sophisticated AI systems. Otherwise, the advances in production go to waste.
The difficulty is that producing results with AI systems alone isn’t enough. The organization must be able to use these results to further business goals.
But the rate of improvement of AI consumption results is slower than the rate of improvement of AI production. Relatively few people produce AI models themselves. Most employees are consumers of the results of those models. Increasing the sophistication of a larger group of users of technology moves slowly, particularly when (by definition) this group of employees isn’t focused on the technology but rather on their business role.
Consumption is necessary because technologies like AI do not work in vacuums. Technology requires business context — which is organization-specific. This context, unsurprisingly, is more difficult to outsource. Instead, many organizations find that it is easier to add technical skills to knowledgeable businesspeople than to add business acumen to knowledgeable technical people. Helping these businesspeople improve their ability to work with AI creates value. According to the 2019 MIT SMR-BCG Artificial Intelligence Global Executive Study and Research Report, organizations that actively help their existing workforces gain AI skills are more likely, by 40 percentage points, to generate value from AI than companies that have not focused on reskilling.
Because improvements in consumption increase more slowly than advances in production, the gap between what an organization can produce and consume can increase rather than decrease as the organization improves its use of technology. While the organization matures in its use of AI in general, consumption still lags production, potentially leading to greater discomfort for the technology users. Ironically, as the organization matures, it faces a growing gap.
Organizations must therefore encourage employees to use their new skills. To improve employees’ comfort with new technologies, Roche Diagnostics uses “innovation theaters” to provide accessible examples of how the organization can use AI. Siemens’ annual internal conference on AI highlights ways that employees can use AI. Both of these examples build on extensive AI skill training. These companies recognize the importance not only of making AI technologies available in their organizations but of reskilling employees to consume them well.
Acquiring the right AI technology and producing results, while critical, aren’t enough. Instead, to gain value from technologies like AI, the company needs to focus on those employees who will consume the AI results that the organization produces.