Progress Toward the Intelligent Enterprise
To deliver on the promise of AI, leaders must focus their efforts on improving their organization’s culture and decision-making capabilities.
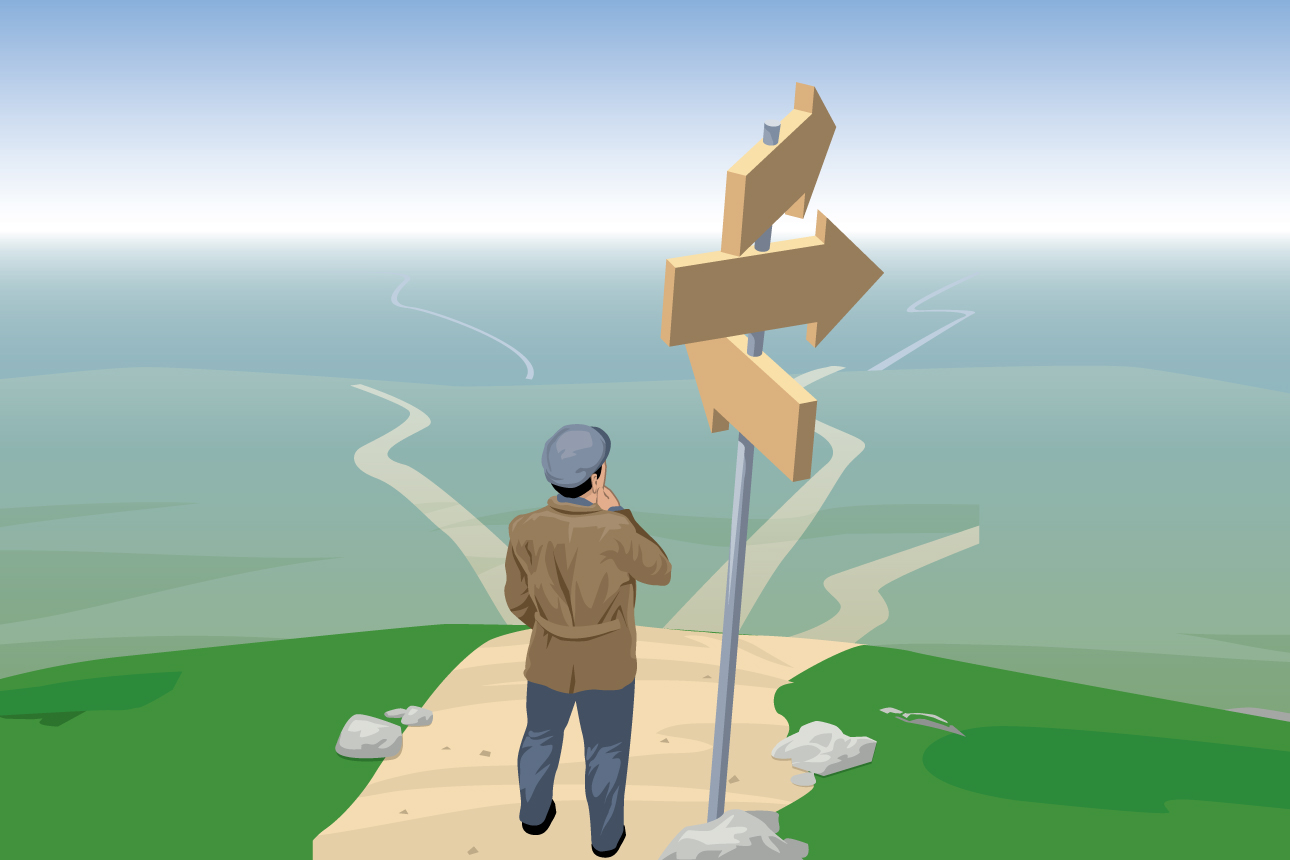
If achieving the intelligent enterprise were easy, everyone would have done it by now. The road to creating, or re-creating, a business optimized by AI to take advantage of machine-assisted decision-making at all levels of the organization is a long one.
Two key questions are, how far along are we on the path toward achieving this vision of future productivity, and are there ways organizations can improve their odds of success?
Companies are now directing billions of dollars globally each year toward AI development, yet more often than not, they’re frustrated by the lack of progress. In fact, only 1 in 10 managers who responded to a recent global survey conducted by MIT SMR and BCG could point to tangible returns. With AI investment expected to more than double to $110 billion by 2024, it’s not surprising that some leaders are asking whether that money would be better spent elsewhere in their organizations.
In one respect, they might be right.
It’s easy to do AI wrong, and it’s very hard to do it right. The organizations that are making the most significant progress on the path toward the intelligent enterprise are the ones that treat the project as a complex systems engineering problem — one that focuses more on adapting the corporate culture than it does on the technology itself.
Avoiding the Path to Frustration
Creating an intelligent enterprise is a fundamentally different undertaking than, say, moving a business to the cloud. For the latter project, one needs to do little more than call up one of the big cloud providers and perhaps hire one of any number of contractors to rewrite applications and train staff members to complete the transition. As long as you perform the due diligence and don’t cut corners on funding, IT projects tend to be straightforward.
The temptation, to the detriment of many companies, is to think of AI development in the same paint-by-number terms. After all, AI development requires IT infrastructure — the more data you have, the more computing power you need to process it. The IT component also happens to be easy to understand, and progress in the field is simple to track. Likewise, the software is more powerful than ever, with an increasing number of robust AI tools being built into common open-source programming packages. Progress toward the intelligent enterprise, however, isn’t reducible to numbers.
That’s because the intelligent enterprise isn’t built around off-the-shelf tools and techniques. The headline-grabbing AI systems are purpose-built and quite narrow in scope. The amazing machine learning systems that can drive a car can’t be used to win a game of Jeopardy! Likewise, the Watson computer that defeated Jeopardy! champion Ken Jennings won’t be driving you to the supermarket anytime soon.
Businesses that are farthest along the path to the intelligent enterprise are the ones that have made a commitment to building teams. They embrace a long-term vision and work systematically to implement a slice of that vision. UPS’s ORION system is a great example of how to do this right.
UPS Navigates a Long-Term AI Strategy
Package delivery giant UPS gained notoriety a few years ago when it announced that its drivers would no longer make left-hand turns when making customer deliveries. Most people don’t give a second thought about whether turning left is the most efficient way to get to their destinations. But the UPS announcement signaled publicly how one of the world’s largest-ever operations research projects had fundamentally changed the company.
UPS’s ORION (on-road integrated optimization and navigation) algorithm system analyzes 18 million U.S. deliveries each day and informs 66,000 drivers how best to get those packages where they need to be at the appointed times. The route calculations take into account multiple constraints to optimize for concerns around fuel efficiency, safety, and distance. The system also can manage wild swings in shipping demands. During COVID-19, for example, U.S. deliveries reached a peak of nearly 40% above pre-pandemic times in May 2020 before settling back to figures that were closer to normal.
These sophisticated capabilities didn’t come cheap. It took a decade for the company to create ORION, which was deployed nationwide in 2016 at a cost of $295 million — and that figure doesn’t count the data-gathering technologies the company had been putting in place since the 1990s. Once the bugs were worked out, ORION’s route optimization algorithm yielded $300 million to $400 million in annual cost avoidance for shareholders, as well as left-turn avoidance for its drivers.
The value proposition was quite obvious once the core project was finished, but that wasn’t true at the start. The development team had to overcome skepticism due to missteps in early phases of the project. However, the team paid a great deal of attention to winning over both the C-suite and front-line managers because they knew the technology wouldn’t matter if it never saw the light of day.
They ultimately won over champions on the executive team who were intrigued by the possibility of creating massive gains in efficiency. These business leaders understood that success required thinking about the complexities of delivery in an entirely new way.
Once it had buy-in, the team focused on working through the problem in a systematic way, decision by decision, rather than by starting with the question, “What technology do we need?” The team developed prototype systems and carefully tested them with select drivers who could provide feedback based on their training and years of experience. The development team knew that transparency would help build trust in the system, which was needed for success — another example of how culture is as important as the technology.
Because UPS used a systems engineering approach to develop the AI system, every step in the project was measured, and the benefits were validated against a series of objective measures. This made it possible to set up dashboards to constantly keep track of ORION’s performance so that executives could monitor progress and, ultimately, the return on investment.
UPS is among the companies farthest along the path to the intelligent enterprise, but it’s still far from reaching that destination. ORION’s ultimate goal is to “optimize an entire network from end to end,” which means, among other things, selecting which drivers are best suited for each delivery area and assisting with all the other decisions involved in designing the best delivery routes. As important as U.S. delivery routes are to the bottom line, UPS is much more than that. It’s a global operation with tens of thousands of storefronts and a fleet of aircraft. Many other critical functions would benefit from AI. What matters is that that company has created the internal systems and culture that can actually deliver on these ambitious projects.
Lessons for the Industry
The UPS experience is instructive because it tells us that there are no shortcuts to building the intelligent enterprise. It’s tempting to see an AI system beat a Go or Jeopardy! champion and think of it as a milestone that brings the intelligent enterprise closer. But it doesn’t.
As amazing as they are, the AI systems that achieve notoriety for besting humans are dealing with a bounded problem — one that has a finite set of rules. Businesses operate in the real world, facing unbounded problems within a universe of effectively infinite possibilities. To give one example, no business had COVID-19 and lockdowns in their 2020 business plans. That’s the kind of surprise that a chess-playing program doesn’t need to anticipate.
Progress toward the intelligent enterprise requires leaders to concentrate efforts on improving their organization’s decision-making capabilities. This can’t be done by merely assembling the brightest minds in one particular field, like computer science or engineering. To the contrary, it requires collaboration across a broad set of disciplines, with experts in different parts of the organization working closely together.
To pull off this collaboration, talent and corporate culture have to align in a way that cultivates new ideas. One way to tackle this is by encouraging diversity in hiring so development team members can benefit from the fresh perspectives that come from their team members’ different experiences and backgrounds. To work effectively toward a shared goal, everyone on the team must receive common training on the techniques needed to approach complex problems systematically. This core training allows the liberal arts major, the engineer, the statistician, and the company’s operational experts to draw from a shared foundation as they apply their unique insights to each situation. These cross-functional teams are ideally suited to tackle ever-changing problems and circumstances as they arise.
As the decades-long experience of UPS demonstrates, making this happen isn’t easy. There is a natural internal resistance to the idea of reinventing everything about an organization from the ground up, and without a culture that will allow this to happen, change is impossible. Overcoming this hurdle is worth it, because the intelligent enterprise can reassign responsibilities for mundane, repetitive tasks that require memory and calculation to AI, freeing humans to focus their efforts on the creativity and judgment at which we excel.