AI on the Front Lines
AI progress can stall when end users resist adoption. Developers must think beyond a project’s business benefits and ensure that end users’ workflow concerns are addressed.
Topics
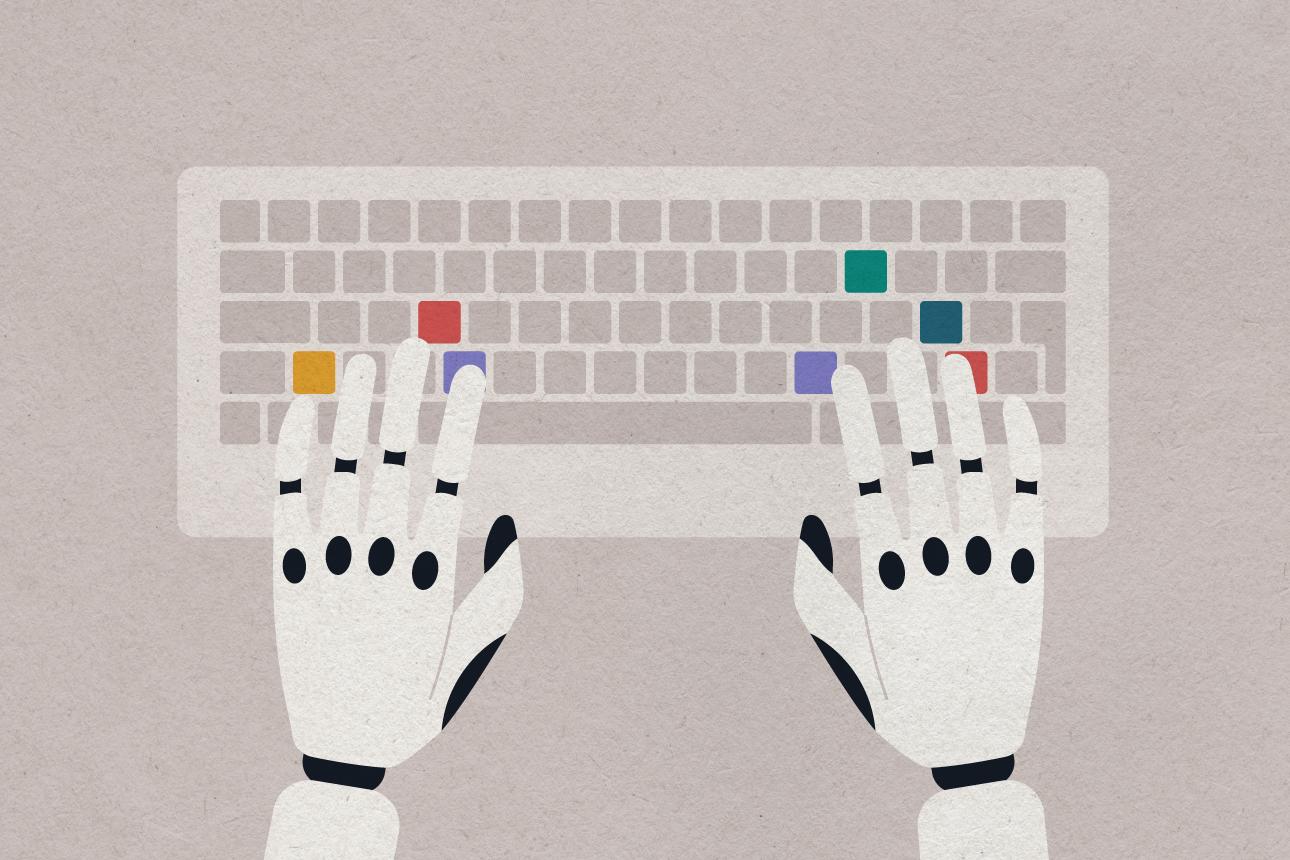
It’s 10 a.m. on a Monday, and Aman, one of the developers of a new artificial intelligence tool, is excited about the technology launching that day. Leaders of Duke University Hospital’s intensive care unit had asked Aman and his colleagues to develop an AI tool to help prevent overcrowding in their unit. Research had shown that patients coming to the hospital with a particular type of heart attack did not require hospitalization in the ICU, and its leaders hoped that an AI tool would help emergency room clinicians identify these patients and refer them to noncritical care. This would both improve quality of care for patients and reduce unnecessary costs.
Aman and his team of cardiologists, data scientists, computer scientists, and project managers had developed an AI tool that made it easy for clinicians to identify these patients. It also inserted language into the patients’ electronic medical records to explain why they did not need to be transferred to the ICU. Finally, after a year of work, the tool was ready for action.
Fast-forward three weeks. The launch of the tool had failed. One ER doctor’s comment that “we don’t need a tool to tell us how to do our job” is typical of front-line employees’ reactions to the introduction of AI decision support tools. Busy clinicians in the fast-paced ER environment objected to the extra work of inputting data into a system outside of their regular workflow — and they resented the intrusion on their domain of expertise by outsiders who they felt had little understanding of ER operations.
Similar failed AI implementations are playing out in other sectors, despite the fact that these new ways of working can help organizations improve product and service quality, reduce costs, and increase revenues. End users often resist adopting AI tools to guide decision-making because they see few benefits for themselves, and the new tools may even require additional work and result in a loss of autonomy.
Such conflicting interests between targeted end users and top managers or stakeholders in other departments around technology implementations are not new. Yet this problem has become more acute in the age of AI tools, because they are predictive, prescriptive, and require a laborious back-and-forth development process between developers and end users.
How, then, can AI project leaders increase end user acceptance and use of AI tools? Our close observation of the design, development, and integration of 15 AI decision support tools over the past five years at Duke Health suggests a set of best practices for balancing stakeholder interests. We found that in order to increase end user acceptance and the use of AI decision support tools, organizational and AI project leaders need to increase benefits associated with tool use, reduce the labor in developing the tools, and protect autonomy by safeguarding end users’ core work.
We gathered data on the challenges that AI tool implementation presented to hospital managers, end users, and tool developers, focusing particularly on the decision support tools that were successfully implemented. Although this research focused on the implementation of AI decision support tools in health care, we have found that the issues and dynamics we identified are also present in other settings, such as technology, manufacturing, insurance, telecommunications, and retail.
The Roots of End User Resistance
The disconnects between what AI project teams hope to implement and what the end users willingly adopt spring from three primary conflicts of interest.
1. Predictive AI tools often deliver the lion’s share of benefits to the organization, not to the end user. The predictions provided by AI tools allow for earlier interventions in an organization’s value chain, with the potential for both the organization and downstream stakeholders to improve quality and reduce costs. However, there are often no direct benefits to the intended end users of the tools, as in the case above, where ER clinicians were asked to use a tool that produced benefits for ICU clinicians.
A similar situation occurred at an online retailer developing an AI tool to flag inbound job candidates whose resumes matched a profile based on employees who had been successful in the organization in the past. HR sourcers, the targeted end users for the tool, often neglected these inbound candidates in favor of outbound search through platforms such as LinkedIn, because they sought to attract a large number of candidates with difficult-to-find technical skills and few inbound candidates had the required skills. Yet inbound candidates were more likely to accept job offers than were candidates sourced through other means, so the tool would benefit the organization as a whole, as well as HR interviewers downstream from the sourcers.
2. AI tools may require labor by end users who are not the primary beneficiaries of the tools. AI tools require a laborious back-and-forth process between developers and end users. Technology developers have long engaged in user-centered design, using task analysis, observation, and user testing to incorporate the needs of end users. But AI tools require a deeper level of engagement by end users.
Because a large volume of high-quality data is required to build an AI tool, developers rely on end users to identify and reconcile data differences across groups and to unify reporting methods. Developers also rely on end users to define, evaluate, and complement machine inputs and outputs at every step of the process, and to confront assumptions that guide end user decision-making.
In cases where downstream stakeholders or top managers are the primary beneficiaries of an AI tool, end users may not be motivated to engage in this laborious back-and-forth process with developers. For example, ER doctors were not interested in contributing their time and effort to the development of the tool for identifying low-risk heart attacks.
Researchers at Oxford University found a similar problem in a telecommunications company developing an AI tool to help salespeople identify high-value accounts.1 While top managers were interested in providing technical expertise to the salespeople, the salespeople valued maintaining personal and trustworthy customer relationships and using their own gut feelings to identify sales opportunities. They were not enthusiastic about engaging in a labor-intensive process to design, develop, and integrate a tool that they didn’t believe would benefit them.
3. Prescriptive AI tools often curtail end user autonomy. AI decision support tools are, by their nature, prescriptive in that they recommend actions for the end user to take, such as transferring a patient to the ICU. The prescriptions provided by AI tools allow internal third-party stakeholders, like organizational managers or stakeholders in different departments, to gain new visibility into, and even some control over, the decision-making of targeted end users for the tools. Internal stakeholders such as senior managers were previously only able to establish protocols for action, which end users would interpret and apply according to their own judgment about a particular case. AI tools can now inform those judgments, offer corresponding recommendations, and track whether end users accept those suggestions — and thus they have the potential to infringe on end user autonomy.
For example, once Duke’s tool for identifying low-risk heart attacks had been implemented, when an ER clinician chose to admit a heart attack patient to the ICU, senior managers and ICU clinicians could see what the AI tool had recommended and whether the ER clinician had followed the recommendation. ER clinicians didn’t like the idea of others, who didn’t have eyes on their patients, reaching into their domain and trying to control their decisions.
A study of the use of AI tools in retail found similar dynamics.2 Stanford researchers examined the implementation of an algorithmic decision support tool for fashion buyers. They had historically relied on their experience and intuition about upcoming fashion trends to decide which garments to stock in anticipation of future demand. For example, the buyers in charge of ordering men’s jeans had to make choices about styles (skinny, boot cut, straight) and denim colors (light, medium, dark). The buyers had considerable autonomy and were not used to having the impact of their intuitive judgments explicitly modeled and measured.
Encouraging Front-Line Adoption
We found that in order to successfully implement AI tools in the face of such barriers, AI project leaders need to address the imbalance between end user and organizational value capture that these tools introduce. In practice, that means increasing the end user benefits associated with tool use, reducing the labor in developing the tools, and protecting end users’ autonomy by safeguarding their core work. (See “Overcoming Resistance to Front-Line AI Implementations.”)
1. Increase end user benefits. End users will be more likely to adopt tools if they perceive a clear benefit for themselves. AI project leads can use the following strategies to help make that happen.
Identify the end users’ “rock in the shoe.” Even as AI tool developers keep organizational goals in mind, they also need to focus on how a tool can help the intended end users fix problems they face in their day-to-day work — or adjust to new workload demands that result from using the tool. For example, when Duke cardiologists asked project team members to build an AI tool to detect patients with low-risk pulmonary embolism (PE) so that the patients could be discharged to an outpatient setting rather than being treated in a high-cost inpatient setting, project team members immediately reached out to the ER clinicians who would be the actual end users of such a tool. The PE project team members learned that the “rock in the shoe” for ER clinicians was rapidly preparing low-risk PE patients for discharge from the hospital and ensuring that they would get needed outpatient care.
AI project leaders attempting to implement the HR tool for screening inbound candidates used this same strategy of identifying the pain point for HR sourcers. The developers learned that HR sourcers couldn’t schedule interviews for candidates as quickly as they would like because the downstream interviewers didn’t have the required bandwidth.
It may seem obvious that AI leaders should focus on how a tool can help the intended end users fix problems they face in their day-to-day work. So why do AI project leaders often fail to do it? Because the people who approach these leaders in the first place, and who provide the resources for tool development, are often the top managers or downstream stakeholders who stand to gain the most from the AI tool. Project leaders often see them as the primary customers and can lose sight of the need to get the intended end users on board.
Develop interventions that address the end users’ problem. Introducing the PE tool at Duke threatened to exacerbate ER clinicians’ problem that there was no easy way to ensure that low-risk patients could easily and reliably be scheduled for outpatient follow-up once they were identified. Once team members learned this, they began to focus on how ER clinicians could easily get these patients follow-up appointments at clinics.
Similarly, developers of the HR screening tool noted that HR talent sourcers had a hard time scheduling interviews for the candidates flagged by the tool. So developers considered how to increase the bandwidth of HR interviewers and wound up suggesting engaging a provider of pre-interview screening services to decrease the workload of the current HR interviewers.
Increase incentives for end users to accomplish outcomes the AI tool is designed to improve. End users who are expected to use a new AI tool to guide their decision-making are often not measured and rewarded on the outcomes the tool is designed to improve. For example, ER clinicians at Duke were measured based on how well they identified and treated acute, common problems rather than how well they identified and treated rarer problems like low-risk PE. Team members worked with hospital leaders to revise the incentive system so that ER clinicians are now also measured based on how well they identify and triage low-risk PE patients.
Similarly, the top managers hoping to implement an AI tool for candidate screening in our earlier example recognized that they would need to change incentives for end users to accomplish the outcome the AI tool was designed to improve. When HR staff members used the AI tool, they would be seen as less productive if evaluated only on traditional performance measures such as total number of candidates sourced with difficult-to-find technical skills. Managers realized that they would need to adapt their evaluation and reward practices so that employees had incentives not only to source a high number of candidates with difficult-to-find skills but also to source a high number of candidates who eventually accepted job offers.
Of course, AI leaders can’t easily increase incentives for end users to accomplish the outcomes the AI tool is designed to improve, because the stakeholders who stand to gain the most from the tool are often not the people who manage performance and compensation for the targeted end users. AI project leaders often need to gain the support of senior managers to help change these incentives.
2. Reduce end user labor in the design, development, and integration of AI tools. There are a number of ways that AI development teams can minimize the extent to which they call on end users for help.
During tool design, minimize end user labor associated with constructing relevant data sets. The data used to train AI tools must be representative of the target population. This necessitates that the volume of training data be large, but assembling such data and reconciling differences across data sets is very time-consuming. AI project leaders can minimize end user labor associated with such work by engaging third-party stakeholders in data construction. For example, Duke project team members worked on an AI tool to increase early detection of patients at high risk of advanced chronic kidney disease (CKD). Data for the tool needed to be drawn from both electronic health records and claims data, and the two data sources were not consistent with each other. Instead of burdening the intended end users of the tool (primary care physicians, or PCPs) with data-cleaning tasks, the team validated the data and normalized it across sources with the help of nephrologists (physicians with kidney expertise), who were the primary beneficiaries of the tool.
AI project leaders can also start with a good-enough AI tool that can be trained using currently available, often smaller data sets. For example, an AI leader developing a tool to help salespeople in a manufacturing organization identify potential high-value accounts wanted to minimize end user labor associated with assembling relevant data sets. Rather than asking salespeople to take the time to better log data related to the different milestones of the sales process (such as lead, qualified lead, and demo), the AI team first built a system with models that were good enough to use but required a smaller amount of training data and thus less data preparation by the salespeople.
During tool development, minimize end user labor associated with testing and validation. Once an initial AI tool has been built, development teams need to engage in a time-consuming back-and-forth process with end users to help test and validate the tool’s predictions and modify the tool to improve its real-world utility. This work can be minimized by engaging third-party stakeholders in the reviews. For example, project leaders developing the AI tool to identify the best sales leads in a manufacturing organization enlisted the head of process improvement rather than the salespeople to help with the initial evaluation of the tool. The head of process improvement helped them identify a success metric of conversion rate — the percentage of potential leads that later became customers. He also helped them do an A/B test comparing the conversion rate for sales leads identified by the tool versus those identified in the regular sales process.
AI project leaders can also frequently do more to help end users more easily evaluate models. For example, Duke project team members developing the tool to detect CKD found that end users had difficulty determining the risk score threshold for considering patients to be at high risk. Project team members used interactive charts to help them see what percentage of the patients with a certain score eventually developed CKD. This allowed the end users to more easily set thresholds for high-risk versus medium-risk patients.
During tool integration, minimize end user labor associated with tool use. Attention to simplifying the user interface and automating related processes can help reduce users’ sense that a tool is loading them up with extra work. One rule of thumb is to never ask the user to enter data that the system could have automatically retrieved. It’s better still if you can actually predict what the user will want and pre-stage the interface so that it is available to them.
In another example involving an AI tool for candidate screening, researchers at the Kin Center for Digital Innovation at Vrije Universiteit in Amsterdam found that developers first made it easier for HR recruiters in a consumer goods company to use the tool by color-coding how well a given candidate matched against employees who had succeeded in the organization in the past. Records of candidates with a match of 72% or higher were colored green, and those below were colored orange.3 Eventually, developers automated the process even further so that recruiters could click a button and the tool would automatically filter out all candidates with lower predicted success.
Another tactic is to reassign some of the additional work required for tool use, if possible. For example, Duke physicians targeted for use of the kidney disease tool suffered alert fatigue, because they were receiving many other automated clinical decision support alerts as well. Duke project leaders reduced the number of physician alerts from the tool by creating a new clinical position and assigning that person to use the tool to remotely monitor all Duke PCPs’ patients — over 50,000 adults. When the tool flagged a patient as being at high risk of CKD, that clinician prescreened the alert for the PCP by conducting a chart review. If the clinician determined that the patient was indeed at high risk of CKD, they sent a message to the PCP. When the PCP received this message, if they agreed that the patient was likely to have CKD, they referred the patient for an in-person visit to a nephrologist.
3. Protect end user autonomy. Humans value autonomy and gain self-esteem from the job mastery and knowledge they have accrued, so it’s natural that users may feel uneasy when AI tools allow stakeholders from outside of their domain to shape their decision-making. Successful AI implementations require sensitivity to how they may affect end users’ relationship with their work. Developers can attend to it in the following ways.
Protect the tasks that end users see as core to their work. When Duke project team members developed an AI tool to help better detect and manage sepsis, an infection that triggers full-body inflammation and ultimately causes organs to shut down, many of the ER clinicians targeted as end users of the tool pushed back. They wanted to retain key tasks such as making the final call on the patient’s diagnosis and placing orders for required blood tests and medications. The project team configured the AI tool so that its predictions did not infringe upon those tasks but did assist ER clinicians with important tasks less valued by the clinicians.
In the case of the fashion buyers, the tool developers learned that buyers wanted to maintain what they saw as creative or strategic tasks, such as deciding what percentage of their overall jeans purchase should comprise boot cut styles or red denim. The project team configured the tool so that if the buyer’s vision was to have red denim, the buyer could add this as an input to the tool’s recommendations so that the red denim order was filled first.
It may seem self-evident that developers should avoid creating AI tools that infringe on the tasks that end users see as core to their work, but AI project leaders may fall into this trap because intervening around core tasks often promises to yield greater gains. For example, in one retail organization, developers initially built a tool to inform fashion buyers’ decision-making. Suboptimal decision-making at this stage had two effects: lost revenue opportunities from not stocking the right products to meet demand, and lost gross margin from buying the wrong product and subsequently having to mark it down. However, fashion buyers rejected the tool, so developers pivoted and went to the other end of the process — developing a tool that helped store merchants decide when and how much to mark down apparel that was not selling. This tool enabled much less value capture, because it focused only on the final phase of the fashion retail process. However, smart AI leaders have learned that intervening around a circumscribed set of tasks with a tool that actually gets implemented is a lot more useful than intervening around end users’ core tasks with a tool that delivers more value in theory but isn’t used in practice.
Allow end users to help evaluate the tool. Introducing a new AI decision support tool often requires replacing a current tool that may be supported by targeted end users with a new tool that threatens to curtail their autonomy. For example, the AI-based sepsis tool threatened the autonomy of the ER clinicians in a way that an existing rules-based tool for detecting sepsis did not. To protect end user autonomy, project team members invited key stakeholders who had developed the tool currently in use and asked for their help designing an experiment that would test the effectiveness of the new tool.
Researchers at Harvard Business School found similar dynamics in their study of a retail organization that developed an AI tool designed to help fashion allocators decide how many of each particular shoe size and style to ship to which store.4 The visibility that the tool gave to managers outside of the process had the potential to threaten the autonomy of the allocators in a way that the existing rules-based tool did not. To protect autonomy, project team members enlisted the fashion allocators in designing an A/B test to evaluate the performance of the existing tool versus the new AI tool.
Giving target end users a say in the evaluation process makes perfect sense, so why don’t all AI project team leaders do it? Because whenever you involve end users in choosing which areas of their work to subject to testing, they will pick the hardest parts. However, since they are the ones who will need to act on the recommendations, you can’t skip this step.
Involve end users from day one. AI project leaders frequently keep AI tool development quiet in the early stages to forestall expected user resistance. But project leaders who don’t involve users early are much less likely to succeed. Users will resent that they were brought in late, and they will hold a grudge. Even if an AI tool can fully automate a process, end users will need to accept the tool in order for it to work. Successful AI project leaders have learned that involving end users at the outset of the project makes that much more likely.
Behind the glittering promise of AI lies a stark reality: The best AI tools in the world mean nothing if they aren’t accepted. To get front-line users’ buy-in, leaders have to first understand the three primary conflicts of interest in AI implementations: Targeted end users for AI tools might realize few benefits themselves, be tasked with additional work related to development or use of the tool, and lose valued autonomy. Only then can leaders lay the groundwork for success, by addressing the imbalance between end user and organizational value capture that these tools introduce. Success doesn’t arise from big data, sparkling technologies, and bold promises. Instead, it depends on the decisions made, day in and day out, by employees on the ground. To make the promise of AI a reality, leaders need to take into account the needs of those who are working on the front lines to allow AI to function in the real world.
References
1. S. Pachidi, H. Berends, S. Faraj, et al., “Make Way for the Algorithms: Symbolic Actions and Change in a Regime of Knowing,” Organization Science 32, no. 1 (January-February 2021): 18-41.
2. M. Valentine and R. Hinds, “‘Rolling Up the Leaf Node’ to New Levels of Analysis: How Algorithmic Decision-Making Changes Roles, Hierarchies, and Org Charts,” working paper, Stanford University, Stanford, California, May 2021.
3. E. van den Broek, A. Sergeeva, and M. Huysman, “When the Machine Meets the Expert: An Ethnography of Developing AI for Hiring,” MIS Quarterly 45, no. 3 (September 2021): 1557-1580.
4. T. DeStefano, M. Menietti, and L. Vendraminelli, “A Field Experiment on AI Adoption and Allocation Efficiency,” work in progress.