Portrait of an AI Leader: Piyush Gupta of DBS Bank
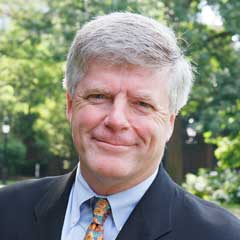
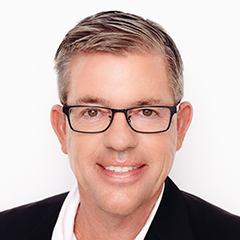
Topics
AI in Action
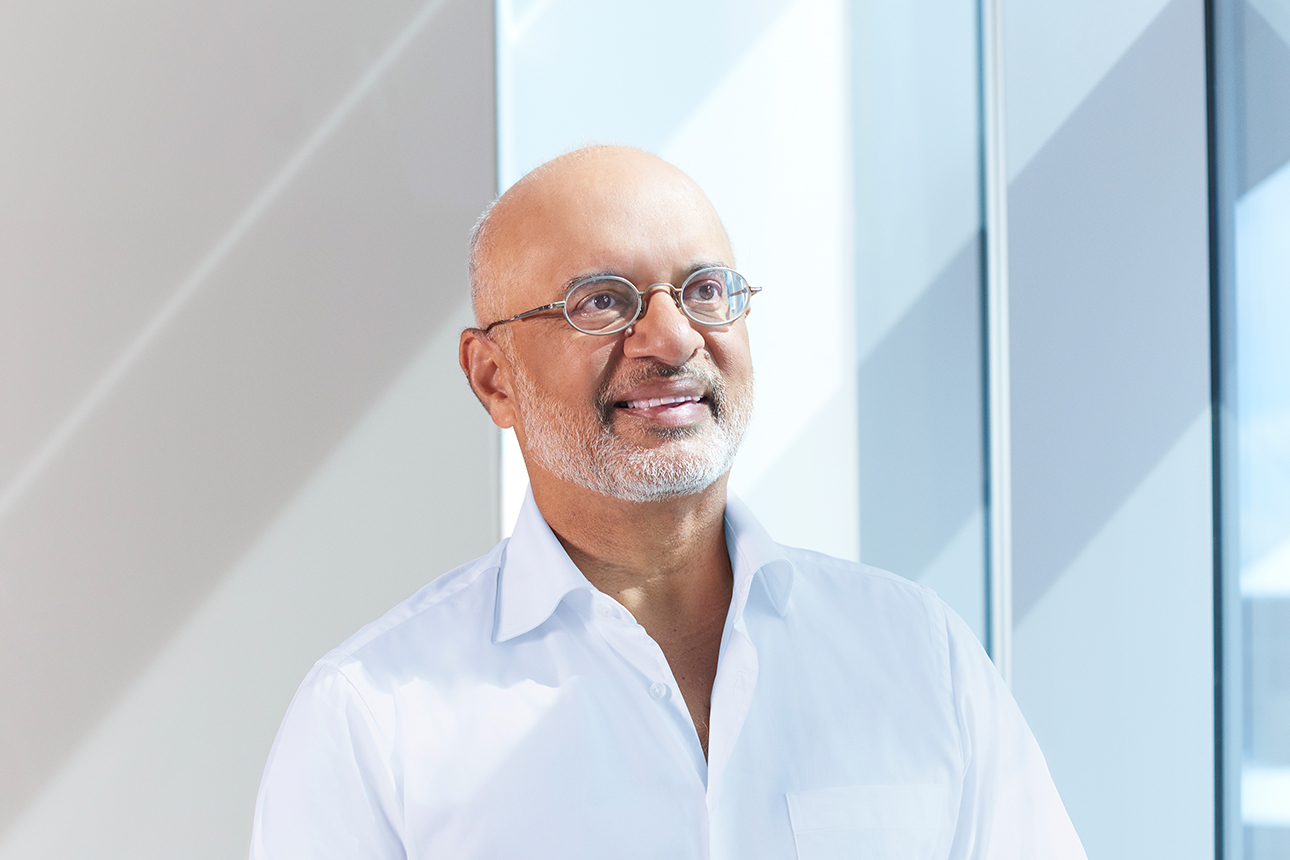
Image courtesy of DBS Bank
It is widely agreed that support from senior leadership is necessary for the successful adoption of artificial intelligence within large organizations. This could perhaps be said of any relatively new technology, but AI stands to have a bigger business impact than most. It is the technology judged by respondents to the 2021 NewVantage Partners technology survey to be the most transformational of all available technologies today.
Piyush Gupta, group CEO of DBS Bank, has been in the banking industry — known for being conservative — for almost 40 years, yet he has managed to create not only a banking and customer service powerhouse out of what was once known as “Damn Bloody Slow,” but also a highly aggressive adopter of artificial intelligence.
Get Updates on Leading With AI and Data
Get monthly insights on how artificial intelligence impacts your organization and what it means for your company and customers.
Please enter a valid email address
Thank you for signing up
Gupta’s efforts and personal passions — and his willingness to push the bank to experiment, even when it might result in short-term failure — powerfully illustrate a senior executive’s ability to lead the effective adoption of a new technology.
Cultivating Broad Experimentation
Gupta arrived at DBS as CEO in 2009, when the company was ranked lowest in customer service among Singapore banks. Today it ranks as one of the best in service and has greatly expanded its footprint throughout Asia through acquisitions and organic growth. It is the largest bank in Southeast Asia and also has a growing presence in China and India. DBS has won multiple global banking awards, including being named Global Bank of the Year by The Banker, Best Bank in the World by Global Finance, and World’s Best Digital Bank by Euromoney — twice.
Gupta told us that his earliest AI initiatives at DBS were failures, albeit instructive ones. He describes them as “signaling tools” for the organization. In 2013, he signed DBS up for an AI lab with the Agency for Science, Technology, and Research (A*STAR), Singapore’s primary public sector research and development organization. DBS signed a three-year contract to explore AI applications with assigned data scientists from both DBS and A*STAR. They worked on half a dozen projects, none of which were successful. But Gupta said that he and the organization learned a lot about the types of AI projects that would be most successful for the bank.
Early on, one of Gupta’s strategies for AI was simply to start with the technology early and try a lot of different things. One of the bank’s key performance indicators was based on conducting a thousand experiments a year, many of them involving data and AI. Gupta revealed these experiments at events he organized in order to promote brainstorming and encourage the bank to think more deeply on how it could deploy AI. AI experiments are, of course, quite common, but not on this scale.
He also “opened up the wallet” to AI experimentation by giving business units and functions the flexibility to hire quasi data scientists to see what they could accomplish. He cited the human resources team as an example of positive outcomes resulting from such an experiment. The head of HR, who had no technical background, created a skunkworks program to identify and pilot AI applications that would help the HR team. The group developed JIM — the Job Intelligence Maestro application — to help the bank’s recruiters hire the right talent for high-volume roles more efficiently. HR also developed an attrition prediction model that enabled the bank to analyze data points such as an employee’s training, compensation, leave patterns, and more to predict the likelihood of their leaving the organization.
Bringing Deep Roots in Technology to Banking
Data is what fuels AI, and many companies have had to implement substantial changes in their data environments to make them suitable for aggressive AI initiatives. What’s not common is for the CEO in a large enterprise to lead the data transformation personally.
Before arriving at DBS, Gupta had been the CEO of Citigroup for Southeast Asia and the Pacific, but his banking roots were in operations and technology. He was a protege of former Citi CEO John Reed, who was perhaps the first global banker to understand the importance of information and technology to the industry, and who led an information-focused transformation of that bank’s back-office and consumer businesses. Gupta led transaction services for Citi in Asia, then rose to regional head for the bank. (He took a brief detour into founding a dot-com business, which he said failed rapidly. To us, that reinforces both his desire for innovation and willingness to miss the mark.)
Gupta credits his interest and ability to drive DBS’s data transformation to his work with Citi, where he participated in the creation of the bank’s first data centers and learned about data architectures.
At DBS, Gupta has led a major transformation in data management. Like many companies, DBS has moved much of its data from traditional warehouses to data lakes; the latter are much cheaper and better suited to less-structured information. In addition, DBS created a new structure for its metadata, cleaned up 80 million incomplete records, developed new protocols for who could access data and what customer data was suitable to capture, and introduced visualization tools to make trends more apparent.
DBS has also created new governance structures for data. For example, it established a Responsible Data Use Committee that examines whether customer-facing information is appropriate to collect and use. The criteria applied are not related only to what is legal, but also to what customers would find acceptable. The bank also follows the mantra of PURE — that data collected should be purposeful, unsurprising, respectful, and explainable. These are all somewhat common data actions for organizations that embrace AI, but it is rare for CEOs to be heavily involved in them.
DBS now employs around 1,000 data and analytics employees, including data scientists, data analysts, and data engineers. Some are in a central group, but more are located in various functions and units around the enterprise. “We have twice as many engineers in technology as bankers,” Gupta noted.
The bank has also trained over 18,000 employees in data skills, creating a company of “citizen data scientists.” About 2,000 employees are proficient in advanced areas of data science and business intelligence, and another 7,000 have been identified to be upskilled in disciplines such as the use of data, analytics, and AI. The bank has been able to create substantial efficiencies with AI in certain areas, such as its customer contact center, using a capable chatbot. However, thus far, increased AI adoption has not resulted in job losses at DBS, in part because people have received training to help them change roles. Gupta acknowledges that while he remains committed to helping his people improve their skills so that they can add value to AI, no one knows just how capable AI will be in the future.
When Gupta was recently looking for new ways to energize people and teach them more about how AI can be used, one person suggested encouraging employees to participate in Amazon Web Services’ DeepRacer League, which uses an autonomous race car simulator to teach machine and reinforcement learning. DBS set a goal of training up to 3,000 employees in 2020 using this approach. Gupta himself competed and, as he put it, “was happy to end up in the top 100 among our people.” But other DBS employees did extremely well, and one was named champion in the AWS DeepRacer League F1 ProAm.
Lessons in AI Leadership
What can we learn about AI leadership from this one example? Gupta’s work with AI demonstrates several lessons that could help leaders at other organizations succeed with AI as well.
- It helps — a lot — to be experienced with information technology. A CEO who doesn’t have a background like Gupta’s can certainly learn enough about AI and related IT infrastructure to be effective, but it will require a good deal of effort.
- It’s important to work across multiple fronts. The specific initiatives in which a leader chooses to get involved will vary across companies, but senior executives are particularly important to signaling interest in the technology, establishing a culture of data-driven decisions, prompting innovation across the business, and motivating employees to acquire new skills.
- Leaders hold the power of the purse. AI exploration is somewhat expensive, and AI development and production deployment is really expensive. AI leaders must devote enough investment to enable both levels of adoption. Note that Gupta opened up the wallet for experimentation in the early days of AI adoption and didn’t require a lot of financial justification.
- It’s valuable for a senior AI leader to become personally engaged with some aspect of AI-focused transformation. Data is always an important issue, though relatively few CEOs will understand it as well as Gupta has. Alternatively, leaders could adopt a particular AI use case and sponsor it to completion.
Gupta made it clear that he is committed to continuing to build DBS’s capabilities in AI, and he believes that the technology will eventually be table stakes in the banking industry. Many other banks have employed AI capabilities from external vendors, but Gupta is committed to building as many AI use cases internally as possible. “We have to have the same capabilities as the digital natives,” he commented. “Then we can continue to innovate and compete with them when we have to.”