What Managers Need to Know About Data Exchanges
The era of big-data silos is fading. Shared data is the future.
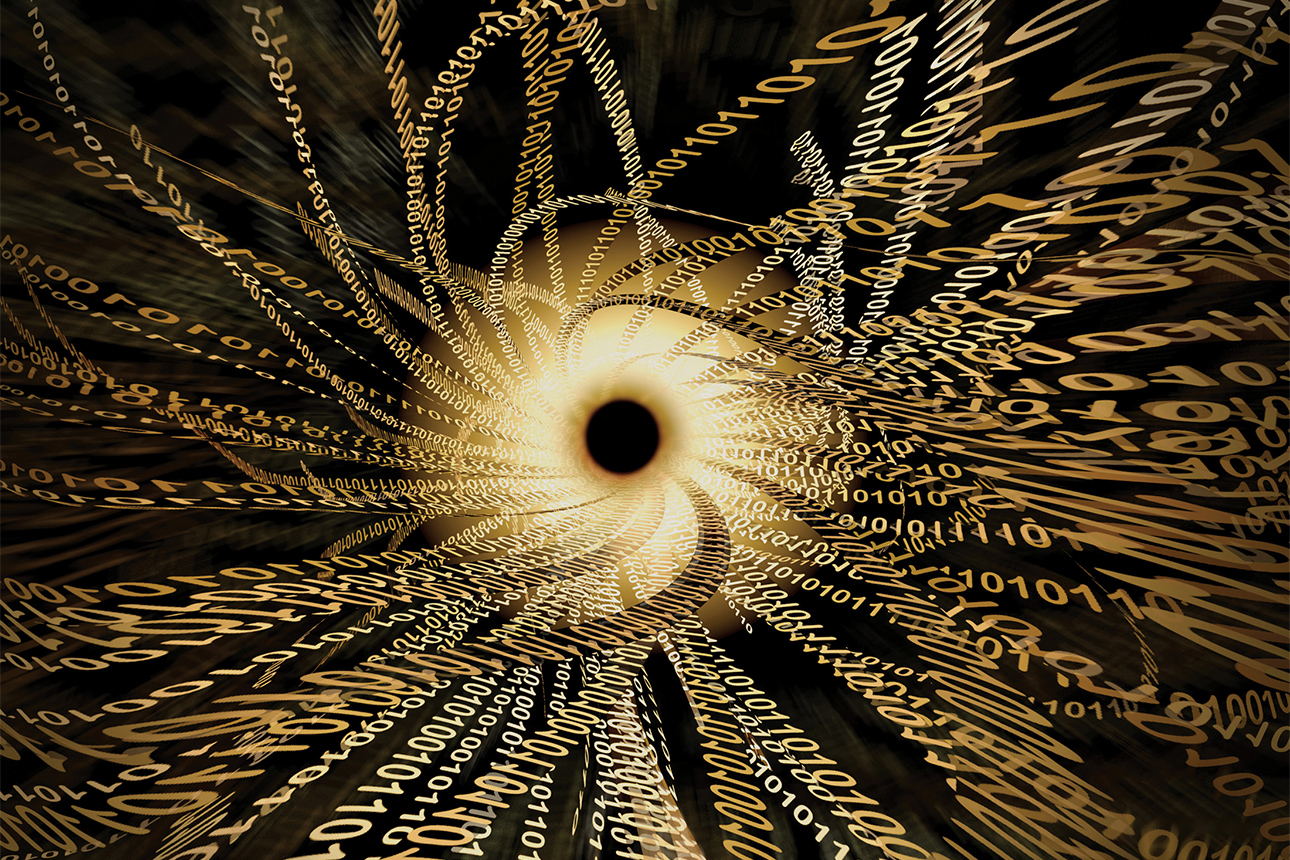
Image courtesy of Jean Francois Podevin/theispot.com
The idea that many businesses rely heavily on data to produce or market goods and services is not new.1 Indeed, even in 2018, four of the six top companies in market valuation — Amazon, Alphabet, Facebook, and Alibaba2 — based their business models on the use of data to optimize advertising. However, data differs greatly from traditional factors of production, such as capital and labor. For instance, to achieve scale, companies need data about large numbers of customers — especially when algorithms are used in advertising and other revenue-generating models. Given that scale, data interacts with personal privacy — even national security — in ways that other factors of production do not. These special attributes of data hinder its efficient and transparent trade in data markets, keep it in closed silos despite its digital nature, and often stop organizations from maximizing its value.
But the conception of big data as a silo managed by single entities is giving way to the notion of shared data. We are interested specifically in data exchanges — shared platforms where data is gathered and curated from many different sources (all the individuals and organizations that voluntarily share it), allowing third parties to gain insights from it. As those insights start to move freely, securely, and confidentially in the market, they will greatly enhance data-based value generation. But for that potential to be realized, managers must become familiar with the unique characteristics of data and with how data exchanges can capitalize on them while mitigating threats.
What Makes Data Unique
Appreciating the full potential of shared data starts with understanding how data is unlike other factors of production:
Data is non-fungible. Distinct units of data can be used differently by the same company. For example, when a business receives, say, $1 of investment, it is irrelevant which specific U.S. dollar of the many in circulation the business receives and whether the investor pays this dollar as one note, four quarters, or 100 cents. That’s because capital is interchangeable. However, should a company receive one unit (say, a megabyte) of data to develop a specific algorithm, not all units of data (health-related data, financial data, geolocation data, and so on) will serve the organization equally well in that effort.
References
1. S. Gandhi, B. Thota, R. Kuchembuck, et al., “Demystifying Data Monetization,” MIT Sloan Management Review, Nov. 27, 2018, https://dev03.mitsmr.io; J. Akred and A. Samani, “Your Data Is Worth More Than You Think,” MIT Sloan Management Review, Jan. 18, 2018, https://dev03.mitsmr.io; and M. Farboodi, R. Mihet, T. Philippon, et al., “Big Data and Firm Dynamics,” Centre for Economic Policy Research, January 2019, https://cepr.org.
2. “The 100 Largest Companies in the World by Market Value in 2018,” Statista, accessed Dec. 6, 2018, www.statista.com.
3. C.I. Jones and C. Tonetti, “Nonrivalry and the Economics of Data,” working paper 26260, National Bureau of Economic Research, September 2019.
4. J. Parra-Moyano and K. Schmedders, “The Liberalization of Data: A Welfare-Enhancing Information System,” SSRN, Jan. 3, 2019, https://papers.ssrn.com.
5. R. Copeland, “Google’s ‘Project Nightingale’ Gathers Personal Health Data on Millions of Americans,” The Wall Street Journal, Nov. 11, 2019, www.wsj.com.
6. A. Garcia, “Google’s ‘Project Nightingale’ Center of Federal Inquiry,” CNN Business, Nov. 15, 2019, www.cnn.com.
7. A. Pentland, D. Shrier, T. Hardjono, et al., “Towards an Internet of Trusted Data,” in “Trust::Data: A New Framework for Identity and Data Sharing” (CreateSpace, 2016): 21-49.
8. “Estonia — the Digital Republic Secured by Blockchain,” PwC, 2019, www.pwc.com.
9. M.T. Islam, S. Karunasekera, and R. Buyya, “dSpark: Deadline-Based Resource Allocation for Big Data Applications in Apache Spark,” in “2017 IEEE 13th International Conference on e-Science” (Auckland, New Zealand: IEEE, 2017), 89-98.
10. A. Hinde, “Journey to the Data Economy,” Data Republic, May 2018, www.datarepublic.com.
11. “Ocean Protocol: A Decentralized Substrate for AI Data & Services,” Ocean Protocol Foundation, April 15, 2019, https://oceanprotocol.com.
12. G. Zyskind, O. Nathan, and A. Pentland, “Decentralizing Privacy: Using Blockchain to Protect Personal Data,” in “Proceedings of the 2015 IEEE Security and Privacy Workshops (SPW ’15)” (San Jose, California: IEEE, 2015), 180-184.
13. Pentland et al., “Trusted Data,” 21-49; and T. Nishikata, T. Hardjono, and A. Pentland, “Social Capital Accounting,” MIT Media Lab, Oct. 17, 2018, www.media.mit.edu.
14. “Endor.coin Protocol: Make Artificial Intelligence Predictions Accessible for All,” Endor, Feb. 18, 2018, www.endor.com.
15. PwC, “Estonia.”
16. Parra-Moyano et al., “Liberalization of Data.”
17. J. Begenau, M. Farboodi, and L. Veldkamp, “Big Data in Finance and the Growth of Large Firms,” Journal of Monetary Economics 97 (August 2018): 71-87.
18. D. Acemoglu, A. Makhdoumi, A. Malekian, et al., “Too Much Data: Prices and Inefficiencies in Data Markets,” working paper 26296, National Bureau of Economic Research, September 2019.
19. A. Pentland, T. Hardjono, J. Penn, et al., “Data Cooperatives: Digital Empowerment of Citizens and Workers,” MIT Connection Science, Jan. 2, 2019, http://ide.mit.edu.
20. D. Walsh, “How Credit Unions Could Help People Make the Most of Personal Data,” MIT Sloan School of Management, July 8, 2019, https://mitsloan.mit.edu.
21. “Business Roundtable Redefines the Purpose of a Corporation to Promote ‘An Economy That Serves All Americans,’” Business Roundtable, Aug. 19, 2019, www.businessroundtable.org.