Demystifying the Intelligence of AI
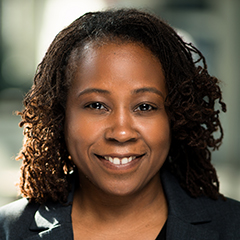
Topics
Column

As artificial intelligence evolves and becomes more intelligent, it’s important for organizations to question its power.
Certainly, AI is being designed to help organizations make jobs more efficient, streamline business processes, and acquire and retain more customers. Companies that haven’t yet incorporated AI are tempted by its operational promises. They’re also tempted by a sense that AI is a tool that will be vital to ensuring competitive advantage, relevance in a rapidly changing world, and not being inadvertently left behind.
But even though AI should be designed to improve human function, it can also hinder it. Near-blind embrace of AI can do nothing for a company — and can even set it back if implemented improperly. AI needs to be demystified, and leaders need to approach it with both optimism and skepticism.
As an executive counselor on the board of the Association for the Advancement of Artificial Intelligence (AAAI) and a board member of the Partnership on AI, I’ve observed best practices by various enterprises and institutions for maximizing the societal benefits of AI. There are three issues that I see most often as trouble points for companies moving toward using AI in their operations: Leaders are unclear about what it means to adopt AI; systems are drawing from too much junky data; and companies aren’t carefully balancing customer privacy and agency with respect to the value returned. These pose major hindrances to AI implementation and can be resolved only when leaders pay close attention to the strategic challenges of bringing AI on, not just as a tool haphazardly slapped onto a process, but as an integrated element of that process.
Three Challenges to AI Implementation
Leaders don’t understand what adoption of AI means. Many companies feel pressured to adopt AI by any means necessary — without thinking through the why and how. I’ve seen many company leaders jump right into gathering data and building models to replace or augment some business function. Very rarely do they systematically plan for, before going full-in, the amount of effort and time they think will be required to invest in the solution, or for what happens if something goes wrong. The metaphor that comes to mind is a fish lured to the next shiny bauble, only to realize too late that the hook will be its last meal.
Get Updates on Transformative Leadership
Evidence-based resources that can help you lead your team more effectively, delivered to your inbox monthly.
Please enter a valid email address
Thank you for signing up
For example, some organizations will adopt new AI functions based on what we call convenience data — data that happens to be available and close at hand. “We want to deploy a new customer service chat bot,” they decide. “Let’s go into our data storage and provide samples from our last N number of customer service calls.” Alas, real-world data, whether from customers, employees, or managers, is not random, is not accidental, and should not be used opportunistically. Data might be clustered by time of day, by customer usage patterns, by geography — by any of an infinite number of parameters. Data that’s not used with discipline can lead to misleading conclusions.
Companies should certainly understand their own data deeply, since it’s their competitive advantage. But they need to approach incorporating AI with their data as a part of their annual strategic plan, in the context of identifying competitors, evaluating their offerings, and developing short- and long-term strategies. AI should be included in that conversation as one solution to identified problems instead of embracing it as the end-all solution.
Like all data operations, AI is subject to the rules of garbage in, garbage out. AI can easily turn valuable data into decision-making mistakes and false truths. The complexity of AI algorithms can make it almost impossible to validate all the possible outcomes over all possible scenarios. As these algorithms begin to be deployed more widely, both integrated within operational processes as well as to enhance customer value, there is an increased risk that they might inadvertently negate their own benefits.
In the lab I direct at Georgia Tech, Human-Automation Systems (HumAnS), one of the issues we examine is what happens to trust when AI systems make mistakes. Over the years, there have been a number of well-known public fiascos that illustrate the problem of garbage in, garbage out. In 2018, The Washington Post teamed up with two research groups to study how well AI voice assistants perform with people with accents living throughout the U.S. The recognition rate was worse for them. In some cases, speech from people with non-native accents had 30% more inaccuracies in recognition. Bias also was initially built into Gmail’s Smart Compose AI feature, which offers suggested text for finishing sentences and replying to emails. A research scientist at Google discovered in early 2018 that when he typed, “I am meeting an investor next week,” Gmail suggested adding, “Do you want to meet him?” — assuming the gender of an investor is male. Google decided to eliminate gender-based replies to avoid offending people by predicting the wrong one. In my mind, that was fixing the problem by pretending it doesn’t exist. Solutions like this are just a bandage, only covering up what you can see and not the underlying problem that might still be present from training on biased data sets.
There are more insidious examples. By now, many people know the story of Tay, a chat bot from Microsoft. Within a day of being deployed, Twitter users engaging with Tay had taught it to develop its own form of racist tweets and improvised commentary. Mind you, a similar China-based chat bot, also by Microsoft, had been released a few years earlier. Over 600 million online users later, the Chinese chat bot hadn’t developed the same problems. I’ll let you make your own judgments about the moral character of Twitter trolls in the U.S., but the point is that if AI actively learns from garbage, it’s going to spew out that same garbage as truth.
These problems are not novel. Even bona fide experts in this domain occasionally admit the difficulties in training new AI algorithms with the data they collect. But this is a challenge that company leaders must respect and pay attention to.
People’s privacy and agency must be carefully balanced with respect to the value returned. Let me first define my terms. Data privacy, which we also call information privacy, covers the relationships between the collection and dissemination of data and the public expectation of privacy. In social science, agency is the ability provided to individuals to act independently and to freely make their own choices.
Given all of the data that companies collect about each of us, their targeting is becoming amazingly accurate, whether it’s for advertising, changing behavior, or making us more efficient. In some cases, it’s also about improving our quality of life, ensuring a better workforce, and enhancing health opportunities. Of course, with the positive aspects of AI and data use, there are also the negative, unethical aspects. A vivid example is when companies use data to identify mental health concerns (potentially a good use) but then scrub that same health data to make a financial profit.
The issue is that, as individuals give up their privacy, companies should honor their decision by ensuring that their agency is maximized. It’s imperative that the value of the AI offering is compatible to the loss of privacy. As I noted in an earlier column about the coming governance of AI, the backlash, if that balance is not achieved, may result in harsh regulations, limiting the freedom of companies to operate.
Leaders Need to Ask More Questions
So is the adoption of AI an expedition doomed for failure? Or will companies do what’s necessary to get this right?
I have two recommendations. First, leaders have to be more considerate about their data. It might not seem like the most efficient use of time to focus on the boring basics like testing, but companies should be open to trying several different AI methods and assessment methods, and not be distracted by the next buzzy thing that AI can be thrown at. When any new hardware is adopted by a company, we expect that a team will be available that understands how it functions, knows what it can and cannot do, and knows how to fix it when it’s broken. If a company doesn’t have this talent, it typically purchases tools or external expertise to fill that gap. The steps for effectively adopting new AI algorithms are no different.
Second, leaders need to be asking the right questions and not be fixated on designing solutions around the data that’s most easily available. Just because a company can build an AI-infused product doesn’t mean it should. If it does, it might be setting itself up to develop a great AI solution that addresses no one’s problems. Different stakeholders and perspectives need to be involved to develop clear ethical standards for the use of AI and its value proposition. The appropriate standards and usage patterns might vary depending on the business unit, or depending on the market segment, but these should be clearly shared.
At the end of the day, we all should be united in our desire to ensure that AI and its use is demystified for all.
Comments are closed.